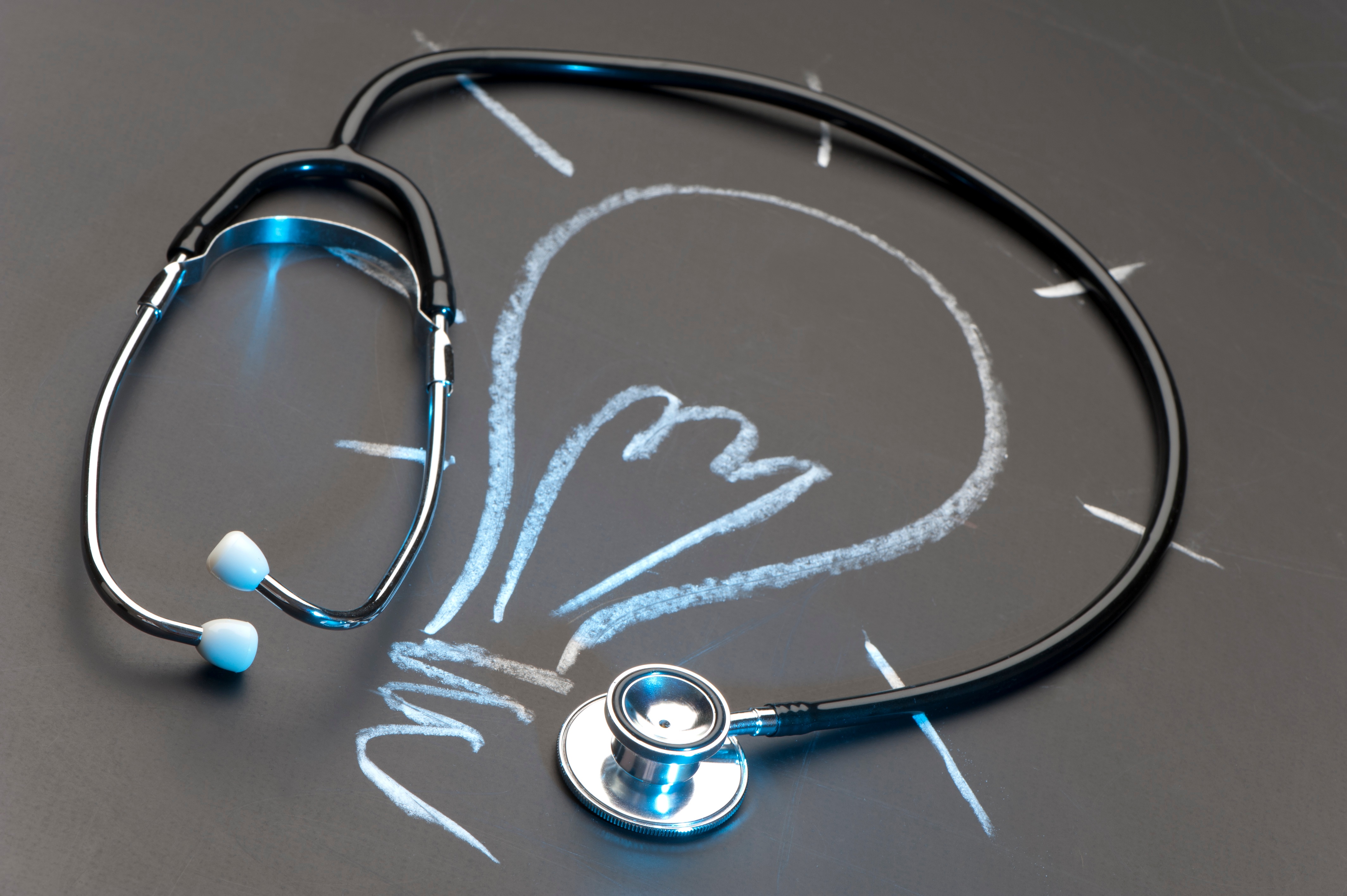
The U.S. health systems ranks 37th out of 191 countries in overall efficiency according to a recent ranking by the World Health Organization. WHO’s calculation includes a variety of indicators including population health, responsiveness, and fairness in financing and financial risk protection1.
The U.S ranks 43rd in life expectancy (79.7 years) behind other developed nations like Japan (84.74), Canada (81.76) and Germany (80.57)2. The U. S. does rank first in one category – total health expenditures, with an average per capita cost of $10,384, nearly twice the comparable country average of $5,1693.
At this point, it is widely accepted that the U.S. healthcare system is sick.
Many healthcare leaders feel the problem lies with the country’s overall approach to healthcare – treating people who are ill. That may sound obvious, but a better strategy might be one that focuses on preventing disease in the first place.
Over 75 percent of healthcare costs are spent treating chronic diseases such as coronary heart disease and type 2 diabetes, conditions that can be prevented in large measure by making significant lifestyle changes. One study of 23,000 people revealed that walking 30 minutes a day, not smoking, eating a reasonably healthy diet, and maintaining an appropriate weight prevented 93 percent of diabetes, 81 percent of heart attacks, half of strokes and more than a third of all cancers4.
Unfortunately, the current U.S. healthcare system is still primarily reactive, focusing on addressing health issues as presented by patients. And unlike other industries who have embraced machine learning and AI to target consumers, healthcare often still takes a “one size fits all” approach to treatment. The combination of reactive care and a standard of care/ population health approach has resulted in a continuously widening gap in quality.
The task of moving from reactive to proactive can be daunting. But recent advances in AI and precision medicine and the growing database of detailed patient data offers a path to a much more effective healthcare system.
Where do you start? Answering these three questions will go a long way towards getting the U.S. healthcare system on the road to recovery.
Where is the value?
Healthcare leaders need to begin by understanding their array of challenges, and determine which offers the most impact in terms of cost and quality if it could be solved.
For example, medication non-adherence is a thorny issue that reverberates across healthcare. In this new value-based paradigm, doctors are being evaluated on outcomes that are often dependent on patients taking their prescribed medicine. If patients fail to take those medications, they are more at risk for adverse events, which increases costs for health plans. Non-adherence also affects biopharma. If patients aren’t using medications properly, it’s difficult to determine the real-world efficacy of drugs and to devise value-based arrangements with health plans.
Non-adherence is a daunting challenge to overcome, especially if patients are not following the personalized care treatment developed specifically to ensure they are receiving the best treatment for them as individuals. By leveraging AI data can be analyzed to ensure patients are engaged in behaviors to improve adherence which ultimately results in better outcomes and reduced costs.
AI adds value to all healthcare stakeholders by determining the optimum intervention along the disease trajectory specific to individual patients. AI platforms can define points of initiation and target patients best suited for an intervention strategy at key “inflection” points. The models are then used to match targeted patients to the optimized interventions thereby matching the right patient, with the right therapy, at the right time.
Do you have the right talent?
To make the most of AI capabilities, providers, health plans and biopharma companies need to employ the best talent possible. A good first step is assessing and leveraging existing resources within their organizations, and ensuring the continued investment in the development of these teams.
Organizations need to move beyond the traditional data and analytics teams to involve talented individuals from multiple disciplines to weigh in on these AI applications. Drawing from clinicians, data scientists and computational experts ensures a multi-faceted approach to these challenges which can provide value across the organization.
Senior executives must also become literate in AI and commit to integrating this technology into their organizations. This requires both a view of how AI can create a strong business differentiation for customers and a more granular understanding of the different types of AI and machine learning approaches. Executives also need to carefully consider how to roll out these pilots and programs – perhaps at first investing in one or two important initiatives that can create a competitive advantage while improving patient outcomes.
Do you have the appropriate data infrastructure?
The healthcare industry is creating 750 thousand terabytes of patient data every day. The key is in parsing that raw data appropriately to solve the specific problems being targeted. To transform that raw data into actionable insights, researchers and data scientists need to determine which measures are most important and which standards can be implemented.
Converting the massive amounts of data into useable information is a crucial component to a successfully implementing AI and requires system interoperability so data can be shared easily across the organization. Once data access is enabled, it must be prepped appropriately to be used in AI/machine learning platforms. Ensuring this data liquidity means creating the appropriate technology ecosystem. This is imperative if organizations hope to derive solutions to their targeted problems.
Moving from reactive to a proactive approach offers a promising path to curing the ailing U.S. healthcare industry. Leveraging the emerging power of AI and machine learning can help make that happen.
Learn more about making the most effective use of intervention programs to improve health outcomes at lower costs by downloading our Intervention Optimization overview.
[1] Measuring Overall Health System Performance for 191 Countries, Ajay Tandon, Christopher JL Murray, Jeremy A. Lauer, David B. Evans, GPE Discussion Paper Series: No. 30, EIP/GPE/EQC, World Health Organization.
[2] Life Expectancy for Countries, CIA World Factbook, 2015.
[3] How does health spending in the U.S. compare to other countries? By Bradley Sawyer and Cynthia Cox, Health System Tracker, Peterson-Kaiser, February 13, 2018.
[4] Change your lifestyle, reverse your diseases, by Dean Ornish, Special to CNN, March 16, 2013.