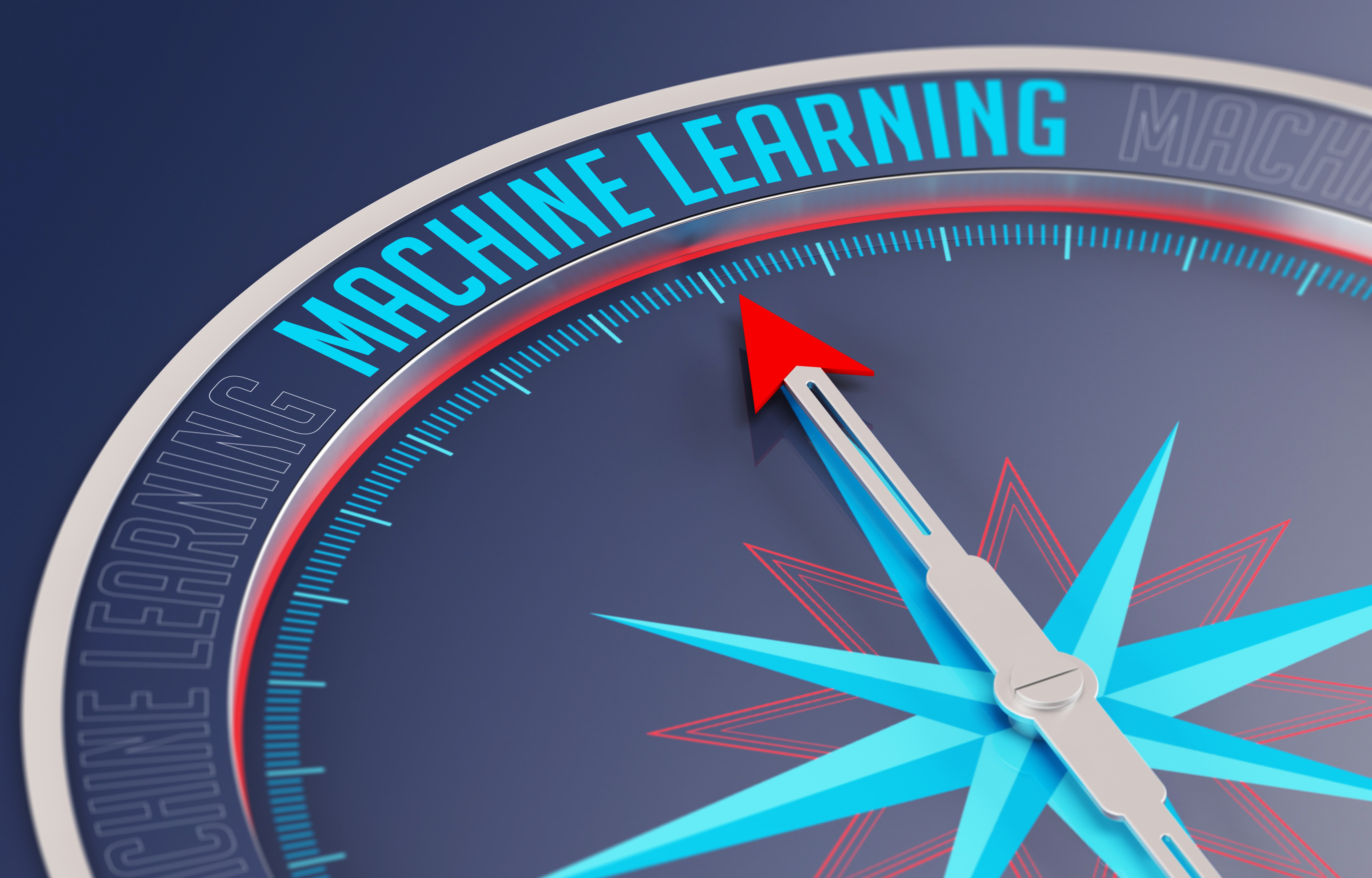
Imagine if we could approach healthcare with the precision that retailers like Amazon and Netflix use to reach their customers. Imagine if we could grasp the holy grail of precision medicine and harness the ability to match patients with the specific treatment or intervention that is most effective for them as individuals.
How can we scale precision medicine so that every patient is provided with a personally optimized treatment plan? How can we turn data into models of disease progression and drug response, so we can discover novel biomarkers and accelerate drug discovery and development?
We no longer need to imagine. These questions can be answered through the application of causal machine learning, a powerful type of artificial intelligence that allows data scientists to glean insights directly from all types of data without needing to test hypothesis after hypothesis after hypothesis.
The power of causal machine learning allows us to apply sophisticated algorithms directly to massive and complex data and accelerate the discovery of cause-and-effect relationships. This then enables health care organizations to answer questions about underlying causes as well as run simulations that answer questions like “what will happen if…?”
Artificial intelligence is revolutionizing healthcare in ways not possible in the not so recent past. Some are calling it the industrial revolution of our time. Here are three ways causal machine learning is now propelling the speed of discovery in health care.
- Ability to integrate and analyze massive data sets of all types
Causal machine learning is data agnostic. This is crucial in a healthcare environment where data is becoming readily more available, but not always in a standardized format. Structured EHR/EMR data, claims, prescription, labs, clinical, and demographics data are usually the most prevalent, and many healthcare organizations are now also able to provide valuable genomics data. Novel data types continue to grow exponentially with new technological developments. Natural Language Processing is now unlocking a treasure trove of unstructured data available from clinical reports and physician notes. And the rise of mobile devices and digital health apps are giving rise to unprecedented amounts of patient reported and consumer behavior data.
Causal machine learning integrates all types of data in massive amounts (think millions of data points) - regardless of structure or format - into the analysis. The resulting reverse-engineered models represent a holistic view of the inner workings of the patient’s condition and the ability to run hundreds of thousands of simulations that reveal the drivers of their individual health outcomes.
- Ability to deploy hypothesis-free reverse engineering
Our causal machine learning platform, REFS, enables discovery of unique insights by first reverse engineering an ensemble of models directly from data, without hypotheses based on prior knowledge. It then runs simulations of the models to make patient-specific predictions and identify the key predictors of health outcomes.
Why is this important? Traditional statistical analyses begin with an explicit hypothesis – a specific statement of how variables combine to explain an observation – which is then statistically tested to confirm if the data supports that argument.
However, healthcare outcomes are often the result of behavior that we don’t necessarily understand well. The analytical tools to measure behavior for doctors and patients are limited and because of this we don’t generally understand differences in variation. For example, if we don’t know specifically what is driving an outcome, we, as an industry, tend to hypothesize, hoping that single hypothesis leads to an answer. Causal machine learning goes beyond hypothesis-based analysis and identifies the causal relationships in large datasets to better understand the drivers of behavior.
Hypothesis-free reverse engineering doesn’t abandon hypotheses as much as “frees” them from constraints of manual formulation and examination. This approach allows you to formulate and examine all hypotheses simultaneously which leads to novel discoveries and the rapid determination of what works for whom.
- Ability to rapidly simulate many “what if?” scenarios into value
The number and variety of interventions is growing daily, from new drugs and devices to care management programs and digital health technologies. With this explosion in patient care options, how do we know what works for an individual patient? By reverse-engineering the cause-and-effect relationship, causal machine learning is enabling biopharma, payers, and providers to ask and answer essential questions that will transform healthcare.
Healthcare has been using analytics for a while and can predict answers to certain questions: what is happening, why is this happening, and what will happen if these trends continue. Predictive goes a step further to answer what will happen next. But those aren’t the questions that will revolutionize the way care is delivered. As researchers, scientists, and clinicians, we need to understand WHY something is happening and then know what specific action we can take to improve that outcome for that individual.
Causal machine learning answers the why and what-if questions that health care needs to answer. What if I treat this patient with drug A versus drug B? How does this patient’s outcome change if I perform procedure X versus procedure Y? Or what will happen to a patient’s compliance if I call a patient versus sending them a reminder text message?
You can only answer these questions by understanding the underlying cause-and-effect relationships within data. Causal machine learning enables the optimization and inference of individual patient care through simulating potential futures and illuminating the best path forward. This is how we get to personalized medicine and truly effective healthcare.
Why does this matter?
There are roadblocks to the advancement of artificial learning in the healthcare industry. Healthcare executives are often skeptical of large multi-year investments in analytical data, infrastructure, and tools. It is difficult for these organizations to make the case to put pressing questions on hold while investing in answering complex questions correctly. As a result, most analytics teams struggle to answer the deluge of specific questions both carefully and quickly while improving access and verifying the learnings that come from analytics. Causal machine learning is one specialized area that could be better suited for partnering given its complexity and dynamic nature.
The challenges that healthcare faces will not be solved by algorithms contained within a black box. As scientists, we need the evidence that tells us why. The potential of what artificial intelligence – and especially causal machine learning – can do to help us better understand rich, complex data is just starting to come into focus. The key is transparency in the application of advanced mathematics to address existing problems.
“What if?” questions are the essence of precision medicine and precision healthcare and using causal machine learning to answer them enables us, as an industry, to expand the delivery of precision medicine to provide far better outcomes at far less cost. Perhaps it is our industrial revolution after all.