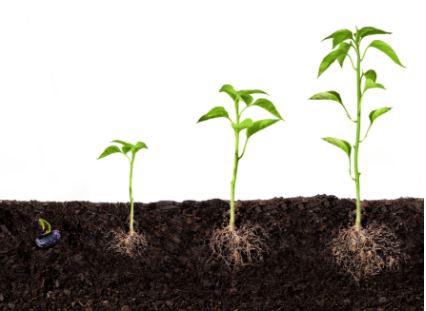
While artificial intelligence and machine learning technologies hold plenty of promise in helping to improve patient outcomes and lower costs in health care, making effective use of these technologies requires expertise and experience in handling massive data sets and the tools that extract the right information to answer healthcare’s most difficult questions.
Julie Slezak, EVP of Clinical Analytics at GNS, highlights the key stumbling blocks she sees most often and how to overcome them. Following are five key challenges. Consider this a giant heads-up to anyone employing artificial intelligence and machine learning in a healthcare setting (and probably lots of other industries as well).
1. Data
“Data is both table stakes and a barrier to entry,” Slezak says. Some companies get stuck trying to find the right data set for the problem they’re trying to solve and get so picky that it effectively derails the project. Others go in the other direction and don’t do enough due diligence on their data, making any results they get questionable.
Part of the issue stems from the differing approaches that IT groups and analytics teams generally take. “IT tends to spend lots of time planning and trying to understand business rules,” she says. “Analytics needs to be fast-paced. It’s rigorous, but not to the point of waiting for something to be fully baked before going forward. That can be a recipe for failure, especially when it comes to machine learning.”
Another issue is understanding how to properly structure and normalize your various data sets. It can take months to get it right. Tools exist that can help, but they take specialized expertise to use effectively.
2. Expertise and talent
This, then, brings up the second challenge, which is expertise and talent. Relatively few analytics professionals and scientists have deep experience with artificial intelligence and machine learning technologies and even fewer also have healthcare experience. So, for now, you have to team data scientists with experts who understand the sorts of questions to ask to get meaningful results for healthcare issues.
3. Getting to root cause analysis
In healthcare, discovery and achieving positive health outcomes often boils down to causality, or what is actually causing something to happen. Understanding causality requires a different effort and specific tools as compared to trying to predict what is likely to happen – which is predictive modeling, a well-understood realm that is far easier to tackle that causal analysis. Getting to causal analysis requires that experts focus in on the specific problem that needs to be addressed, which is where the healthcare expertise comes in.
“How do I take challenges we’ve been answering poorly for some time in healthcare and get to real solutions?” she says. “You have to look for root cause analysis, causality.”
Part of the issue is using the right tool for the right questions, and understanding what kinds of questions can be answered with predictive models versus which require artificial intelligence and machine learning. It can be a fine line between the two. Prediction is about what happens next and trying to anticipate or prepare for that outcome—which patients may be readmitted to the hospital or what patient might be at risk for a heart attack. Causality is about figuring out why something is happening so you can make a decision that may prevent or change the outcome. For instance, understanding why some patients progress faster in Parkinson’s disease so you can target the right biomarkers for drug development to slow or stop that progression. The different between reaction and prevention is the key to understanding prediction vs. causal.
Another opportunity: causal questions must be hypothesis-free. “We answer questions using data as opposed to using hypotheses,” she says; no assumptions allowed.
4. Investment
It takes patience and a long-term commitment to build a truly effective artificial intelligence and machine learning practice. GNS has been at it for 17 years and it was 1997 when IBM’s Deep Blue beat the world chess champion, Garry Kasparov.
Given that reality, not every health care organization will be able to cost-justify or have the luxury of time to build an artificial intelligence / machine learning practice in-house, Slezak says, nor should they. What’s important is to have enough expertise in-house to address issues that you deal with day-to-day. A health insurance company, for example, needs enough analytics capability to be able to answer questions for clients and investors.
Companies are increasingly turning to machine learning to provide better care and become more efficient. Often, they’re better off partnering with another firm that has deep expertise in that area – and that has already made the investment in the technology and capability.
5. Getting to behavior change
Ultimately, artificial intelligence and machine learning technologies may help healthcare companies address what so far has been a difficult problem: getting patients to change their behavior. To date, Slezak says the approach by most healthcare organizations has been to use an old marketing strategy, A/B testing. Try approach X with a certain cohort of a patients and approach Y with another, then go with whatever works better.
But that approach again doesn’t get to causality. “Companies are answering questions for the moment, but they need to get to longer-term, bedrock answers, and the more quickly they get answers the better outcomes they can drive” she says.
For example, it’s known that many diabetics aren’t taking their insulin, yet only some wind up in the hospital. “So, what are we missing? Did they not need the insulin?” Slezak asks. “There are so many things we don’t understand about why people do or don’t do certain things and why they are or aren’t having bad outcomes. Looking at causality in a hypothesis-free manner can be the game changer we are looking for – helping to more quickly discover the relationships and true root causes of important health-related issues.”