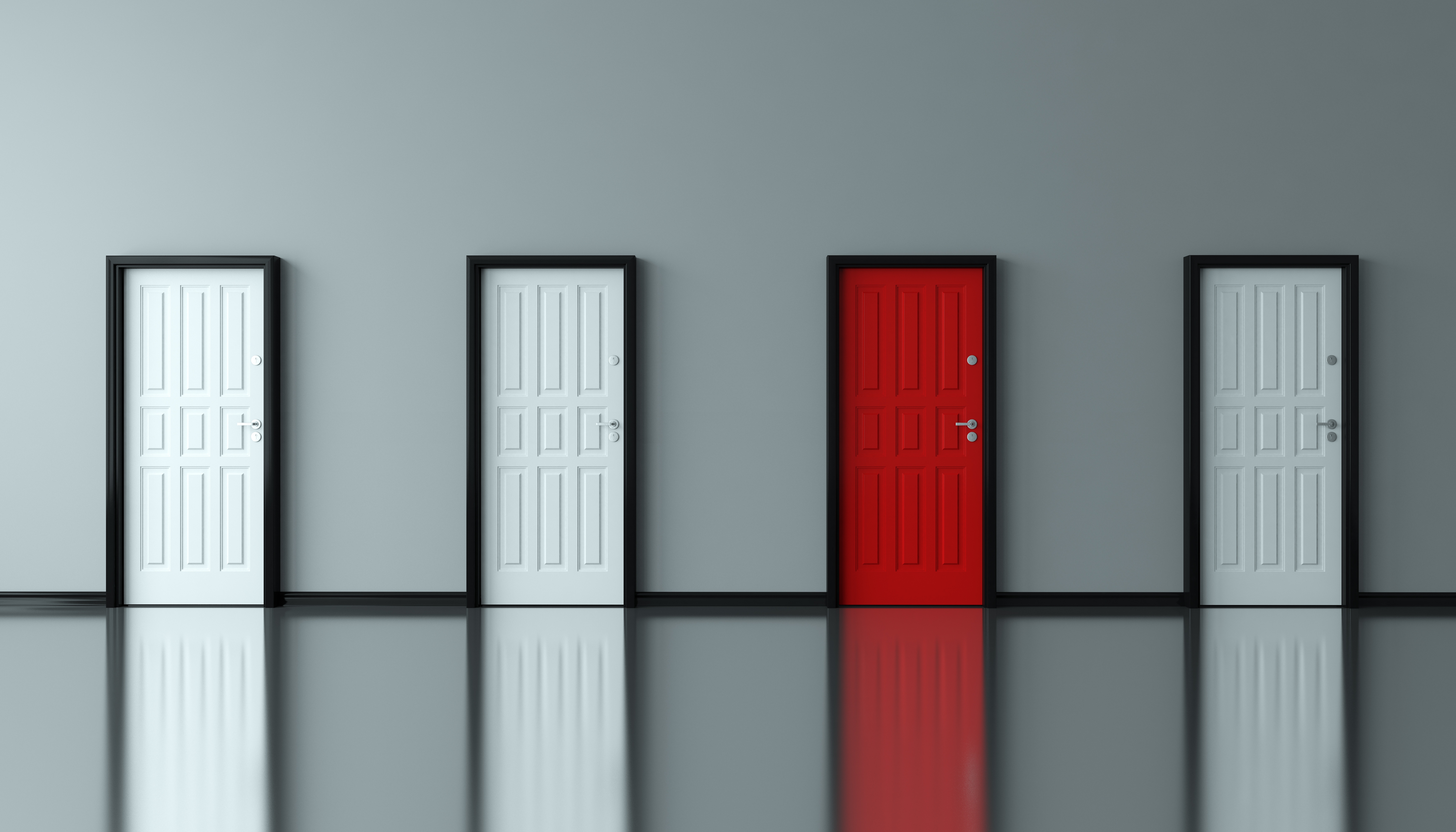
Judea Pearl is not happy.
One of the pioneers of Artificial Intelligence in the 1980’s, Pearl said in a recent interview in The Atlantic that the field of AI is stuck in a world of reasoning by association and probabilistic predictions. Pearl thinks too many people are deploying AI to overcome uncertainty – predicting what will happen next by association rather than leveraging the power of the technology to deal with cause and effect. He goes on to say that AI and machine learning need to move more aggressively to evaluate interventions and causal models to gain true value1.
Healthcare certainly benefited from early AI efforts that processed data to develop predictions and enabled clinicians to improve diagnoses. However it has become clear since then that predictions based on correlations aren’t enough to truly impact the healthcare system.
Correlations can often lead to insufficient or inaccurate conclusions. This point was clearly illustrated by an observational study on women’s health conducted in the 1990’s that concluded that Hormone Replacement Therapy (HRT) had a beneficial effect in mitigating heart disease. The same statistical view of the data also revealed a protective effect of HRT on homicide rates. When experts re-analyzed the data and adjusted for important confounding factors, they found that HRT actually had an adverse effect on heart disease and no effect on the homicide rate.
The false finding was not a result of incorrect data or faulty statistical analysis, but instead highlighted that observational studies relying on correlation can be misleading if important causal variables are not considered. Getting to the right conclusions is not just about collecting the right data and having the right measures, it’s about going beyond standard statistics to learn about the system that caused the results.
Outcome simulation key to causal learning
Tom Davenport of Harvard University and Babson College describes the steps to optimize and infer causality in his book, Competing on Analytics. He divides analytics users into five categories based on the types of complex business questions they are trying to answer.
Most healthcare researchers have worked their way up from the base of standard reports, to statistical analysis, to forecasting/extrapolation to predictive modeling. That, as Pearl recently lamented, is where most analysis stops, creating predictive algorithms but not moving on to finding the root causes of the “why.”
Getting to the top of the pyramid – optimization and inference – and being able to infer the mechanisms underlying the data in the systems requires a different type of analysis—one that enables the in-silico simulation of future actions. This type of analysis is possible using a powerful form of AI, causal machine learning, that can help determine and optimize treatments for specific individuals. Causal machine learning is unique in its ability to take diverse data—the out of real world actions—and use it to make sense of the environments that created the data. The machine learning models that are created are like maps of how the different variables interact with each other and, once understood, researchers and scientists can simulate cause and effect of future actions.
What does this mean for healthcare
Advancing from prediction to causality and then running simulations at scale eliminates errors that result from simple predictive assertions.
Consider a group of patients suffering from a particular disease. These patients are often looked at and treated as a homogeneous population. But since we know that no patient is average, how can we use causal machine learning to ensure that these patients receive the right treatment for them and their specific disease?
The first step is to gather the enormous amounts of granular data that patients with this given disease create—genomics, genetics, claims, labs, EHR data, and so on. This data, in aggregate, gives causal machine learning the fuel it needs to create mechanistic models of the systems that give rise to the data.
Once researchers have these mechanistic models that showcases relationships between the variables, they can begin to query the models with “what if” questions and run causal simulations to glean the answers. Questions like:
- What is driving a patient’s response to treatment?
- Does a patient respond better to treatment A, treatment B, or some combination of the two?
- What other disease areas might benefit from this treatment?
Answering these types of questions moves beyond general population trends to get to an understanding of the patient, the treatment, and how various interventions may change health outcomes. Leveraging these models can help biopharma companies develop new therapeutics based on targeted subpopulations, understand the value of their drug in the real world prior to launch, or identify an additional population of patients who would benefit from their drug.
These simulations also benefit patients when it comes to care treatment plans. Payers and providers can better understand the possible trajectories of the patients’ disease and establish the ideal intervention points to positively impact the disease progression. The simulations can predict the sequence of interactions and events and enable researchers to discover the optimal intervention-member-time match for clinical outcomes, quality measures, hospital bed days avoided and total cost of care.
Success for the healthcare industry means putting the tools and platforms to create these causal simulations into the hands of not just data scientists but clinicians, researchers, biologists and others responsible for creating new treatments and delivering the interventions as well. Because at the end of day, what’s the point of predicting the future for patients if you can’t understand it or change it?
[1] How a Pioneer of Machine Learning Became One of Its Sharpest Critics, by Kevin Hartnett, The Atlantic, May 19, 2018.