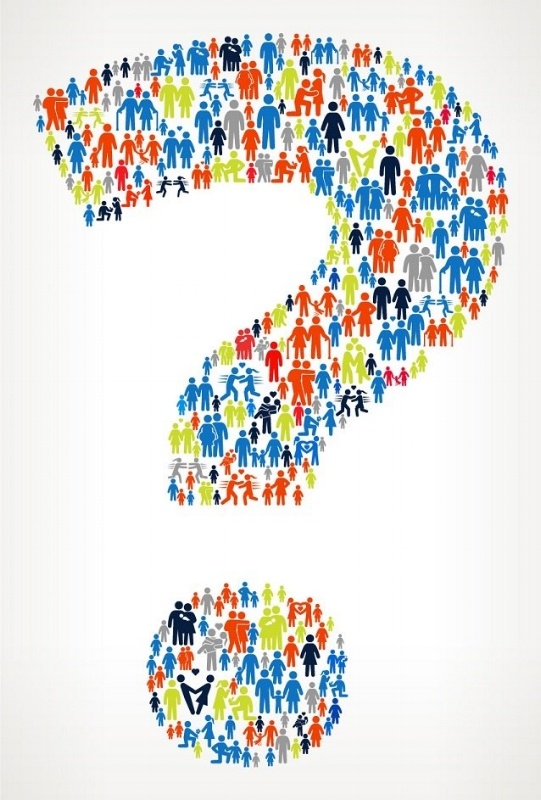
Between 2006 and 2016 there were over 2 million scholarly articles published around the globe. Of those, more than 25 percent – 568,000 – were focused on medicine, more than double the next area of study¹.
Despite the spike in medical knowledge, there is still a struggle to understand why some treatments work while others do not and the reality is that there is still much we don’t know.
In recent years, there has been equal parts hope and hype over Artificial Intelligence (AI) and how to use it to unravel human disease more quickly and efficiently. In a recent article in Forbes, physician/scientist/management consultant David Shaywitz explains that the practice of medicine traditionally has been driven by empirical evidence – experimentation, observation and change. He asserts that the lack of empirical evidence or “mechanistic explanation” is the main reason for the lack of adoption of AI in the healthcare community. And he’s not wrong.
Medicine has used the scientific method practice approach for hundreds of years to form a diagnosis, prescribe a treatment, and then wait to see if there is improvement. There is hesitation to move to an alternate path largely because AI has been presented as a black box, meaning models and algorithms produce results that can’t be clearly explained.
Stakeholders across healthcare are understandably reacting with a “Not so fast. I need to know more” attitude. They want to know more than the “what” of results. They want to know the “why”.
But it’s not quite as black and white as presented. While many forms of AI fail to provide critical information on the way it derives their results, one branch of AI does answer the “why.” It’s called causal machine learning (CML).
Getting to the “Why”
Causal machine learning may be a new concept to some, but it is crucial to the future of healthcare (and if you’re a regular reader of our blog, you are familiar with the term). CML is a powerful form of AI that discovers and explains the cause and effect relationships within data that are driving outcomes. Using the vast amount of diverse data being created and collected today as the fuel, it builds models of diseases and drugs to help researchers, clinicians and care managers understand what works for an individual patient and most importantly, why.
CML has its roots in the field of systems biology, where physics is applied to medicine. It creates causal models of the mechanisms of a system to explain how the system works by leveraging the immense computing power now at our disposal. These models are crucial to create a better understanding of how a disease develops and progresses, or how a drug performs. Simply put, CML constructs in silico models of biology, a sort of blueprint similar to physics models that explain how planes fly, or a circuit diagram that represents how electricity travels.
CML goes a step further than other types of AI by allowing users to interact with a model of a system and ask questions about why something happened and answers the questions of “what will happen if?” This transparency is incredibly important because it allows researchers, drug developers and clinicians to understand the specific variables that are driving disease progression and treatment responses.
Why causal matters in healthcare
CML builds on the mathematical approaches in causality developed by Dr. Judea Pearl. He is credited with the development of Bayesian networks – a mathematical formalism for defining complex probability models and developing the principal algorithms used for inference.
Dr. Pearl uses the example of Simpson’s Paradox in many of his writings on the mathematics of causality to explain why causal is so important in healthcare. Simpson’s Paradox is a statistical phenomenon where it’s possible to draw two different conclusions from the same data depending on how it is divided. Solving this dilemma requires moving outside standard statistics and understanding the causality involved in the situation.
Understanding the underlying causal context – getting to the “why” - of statistics has huge implications in healthcare. The goals of AI in healthcare are much more significant than those for other industries. It’s not about nudging people to buy the latest product or to click on a banner ad to capture their email. When it comes to healthcare, people need to know “why” a particular drug or therapy is being recommended. The magnitude of healthcare decisions and choices make the “why” crucial - as it is someone's health on the line.
So yes, there are types of AI that remain a black box, like deep learning, neural networks and cognitive computing, but those are not the only types of AI being applied to solve healthcare’s challenges.
We are on the precipice of making personalized medicine a reality because of unprecedented progress in the last two decades - the mapping of the human genome, the continual evolving sources of data, more and more cross industry partnerships, incredible computing power and yes, AI. It is incumbent on each of us to understand the different types of AI, what they are best used for and get to the point where drugs are used on those most likely to respond, human lives are improved, and diseases are cured.
[1] Scholarly Publishing in 2016: A Look Back at Global and National Trends in Research Publication, American Journal Experts, 2018.