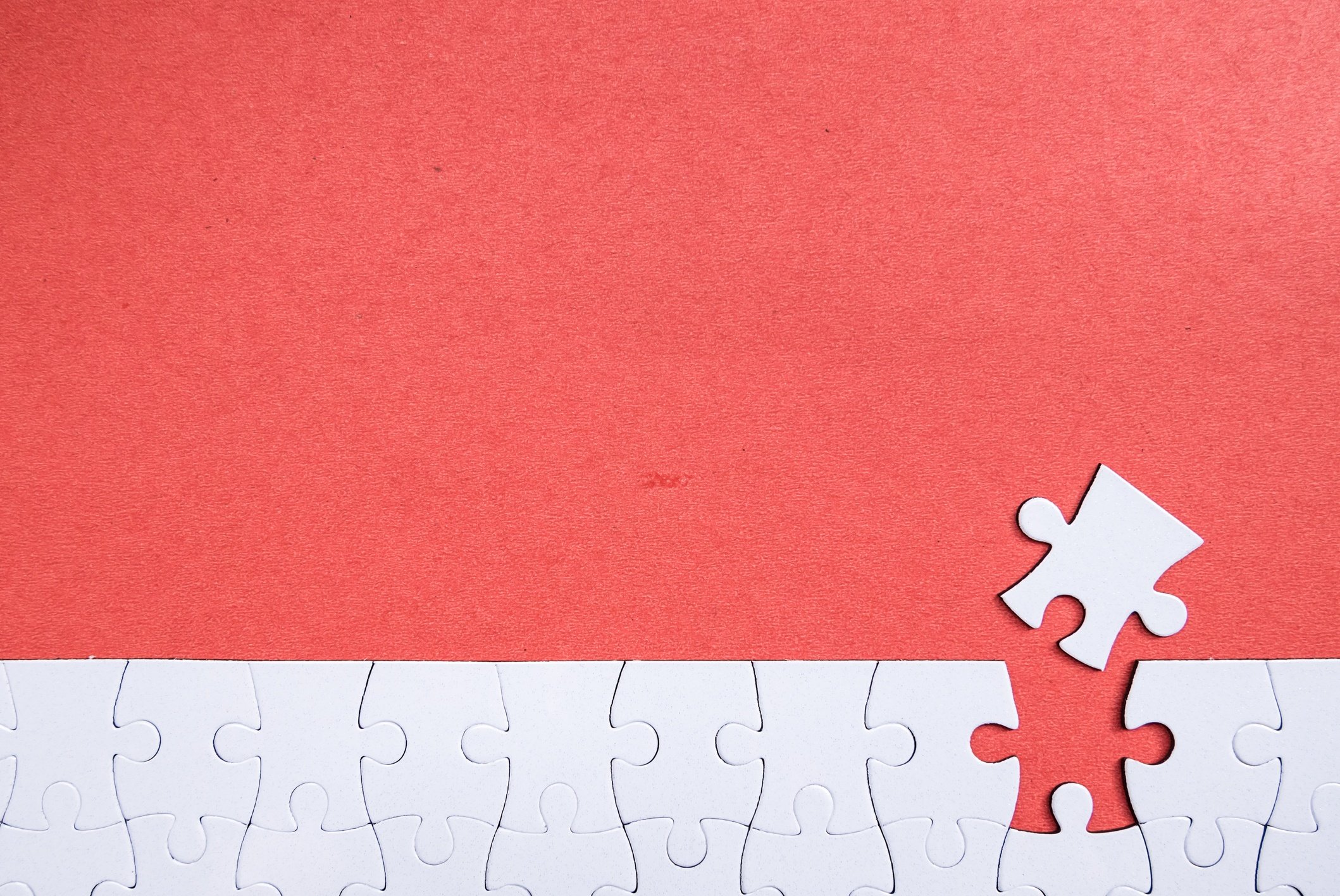
More than 30,000 people a year are diagnosed with multiple myeloma in the United States, making it the second most common type of blood cancer. Researchers have yet to find a cure, in part due to the diverse ways the disease manifests itself, but recent advances in cancer research have made it a treatable disease.
The availability of genomic data and power of artificial intelligence is driving more progress in the understanding of multiple myeloma. Causal machine learning (causal ML) – a powerful form of artificial intelligence – has the ability to reduce the time to study disease and discover new biology from years to months. However, for causal ML to be successful, it needs vast amounts of reliable data. The Multiple Myeloma Research Foundation (MMRF) through its CoMMpass Study has the largest genomic data set of any cancer, enabling a powerful partnership.
A powerful collaboration
CoMMpass is a longitudinal study of over 1,000 patients with active multiple myeloma. The goal is to map each of the patient’s genomic profiles to clinical outcomes and to develop a more complete understanding of patient responses to treatments. It collects genomic variation, gene expression changes, epigenetic changes and clinical outcomes longitudinally across a well characterized population of multiple myeloma patients.
The collaboration is driving the discovery of new drug targets, pathways and new combination therapies by unravelling the underlying complexity of this disease. It is also enabling the discovery of biomarkers that enable the stratification of patients in clinical trials so that drug development can be accelerated and benefit patients faster.
More data, more insights
The collaboration between GNS and MMRF began three years ago, by building models using the CoMMpass study. As more patients were recruited to the study, and more data was collected on existing patients, additional information became available for the technology to find causal relationships. The more data that is added to the platform, the stronger the models become.
As a result of deploying causal ML to the large CoMMpass data set, researchers identified a network of variables potentially driving high-risk status, notably involving cell-cycle regulators CDK1, MELK, and PLK3, known targets of inhibitor drugs.
A second result included identifying a sub-population of patients for whom stem cell transplantation may be particularly effective in treating multiple myeloma. By reverse-engineering models of multiple myeloma patients, looking for connections between specific treatments and outcomes, the CHEK1 biomarker was discovered as a potential biomarker to signify stem cell transplantation effectiveness. Depending on the level of expression of this biomarker, researchers are able to predict which patients may have a positive response to stem cell treatment and which may not. These results were then validated in data collected from an independent phase 3 trial led by the Dana-Farber Cancer Institute with 700 patients and in laboratory experiments.
This use of AI is very different from typical research, where scientists start with a hypothesis and begin testing by changing only one condition, or variable, at a time. The causal ML platform is able to test all hypotheses in the same model. As a result, the CHEK1 biomarker bubbled to the top as an important indicator of stem cell transplant benefit. The modelling began without any idea that CHEK1 had any relationship to the treatment. It only took a matter of months to identify CHEK1, as compared to the “years and years” of testing that would have been required by testing one hypothesis at a time.
The discoveries of the high-risk markers and the CHEK1 biomarker for stem cell transplantation are just the latest in a series of discoveries made possible by the collaboration between MMRF and GNS. Previously, the partnership also identified a number of novel pathways that drove favorable responses to treatments, including pathways of different types of genes.
Precision medicine
There are many beneficiaries to the work being done by the application of artificial intelligence to longitudinal data. Patients benefit from more precise, effective treatment. Health plans gain valuable knowledge of treatments that are both more effective and less costly. Pharmaceutical companies are able to develop more targeted therapies.
Precision medicine is on the horizon and the promise of improving outcomes, extending lives and reducing costs is no longer a pipedream.