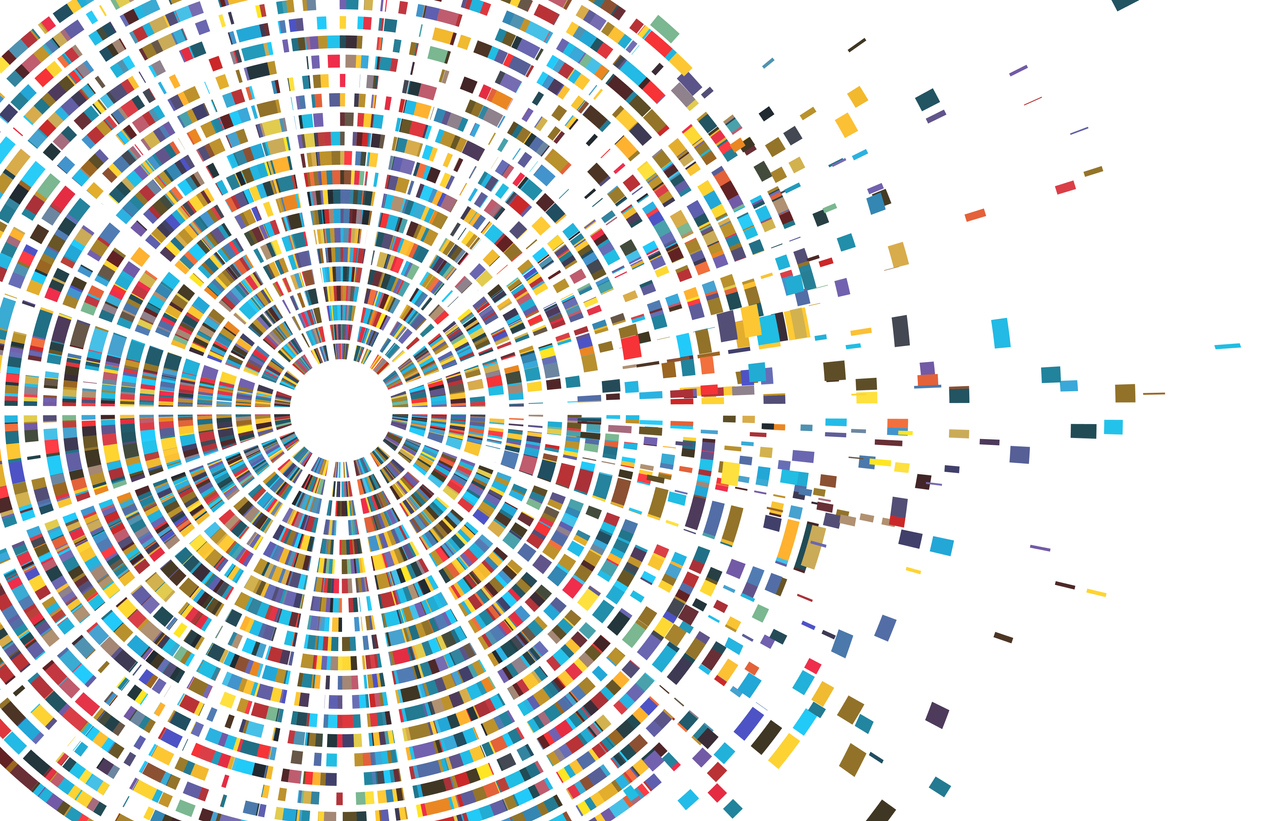
Biomarker-guided precision medicine is increasingly important in drug discovery and development. Pharma products that incorporate biomarkers to support optimized trial design and selection criteria are three times more likely to make it from Phase I to FDA approval than those that do not.[1] Clinical trials that leverage biomarkers for patient stratification to guide their inclusion and exclusion criteria made up about 34% of oncology studies in 2017.[2] Clinical trials costs are also affected by biomarker-driven development. Equally impactful, one study notes that the use of a validated biomarker in patient selection reduced clinical trial risk by 50% and resulted in 27% cost savings.[3]
The positive motivators for biomarker-guided development are clear, but simultaneously, the shift towards value-based care and “pay for performance” formulary structures place a premium on the ability to determine which drugs are effective for which patients. An example of this shift are the agreements reached for evolocumab and alirocumab (cardiovascular drugs) by manufacturers, both of which agreed to reimburse patients or insurers if patients did not show the same degree of improvement on the drug as clinical trial participants. Overall, the movement toward keeping efficacy in mind in formulary placement and drug cost is unavoidable.[4] Biopharma firms will increasingly be asked to shoulder the financial cost of drug treatments that do not identify which patient populations will benefit the most from treatment.
What is Biomarker Driven Drug Development?
The FDA-NIH Biomarker Working Group defines a biomarker as a “defined characteristic that is measured as an indicator of normal biological processes, pathogenic processes, or responses to an exposure or intervention.”[5] In other words, a biomarker is a measurement of some aspect of physiology or disease that correlates with patient prognosis or response to treatment. The idea of a biomarker isn’t new – arguably, it dates back to Hippocrates who noted the relationship between various visible biological manifestations of disease. But many people point to the FDA approval of trastuzumab in 1998 for HER2-positive metastatic breast cancer patients as the advent of biomarker-guided drug development.[6]
Since that time, the biopharmaceutical industry has increasingly focused on identifying biomarkers for response to drug treatment as an integral part of drug R&D. These studies involve three general components: research that searches for potential biomarkers in human tissues or animal models of disease; the development of a reliable test for quantifying the amount or presence of the potential biomarker; and the design and execution of clinical studies that prove the value of the biomarker for identifying patients that benefit from treatment with the drug of interest.
The Data Explosion Powering Biomarker Driven Drug Development
The publication of results from the Human Genome Project in 2001, and its demonstration of the feasibility of large-scale molecular characterization of DNA, was a milestone for biomarker science. The cost of whole genome sequencing has declined precipitously since then, now coming in at approximately $1000 per genomic sample, leading to massive increases in the generation of information for identifying DNA biomarkers. At the same time, other “omics” technologies for measuring other molecular characteristics of human biology (RNA, proteins, lipids, metabolites) have enjoyed widespread adoption in research. The explosion of data that can be generated via multiple ‘”omics” technologies makes it both necessary and attractive to use mathematical methods to sift through the large amount of data to identify possible biomarkers. As one example of how this might be done, patient tumor samples from a clinical trial could be analyzed using omics profiling, and mathematics used to establish which molecular markers correlate with longer survival for a patient receiving a cancer drug.
The Need for AI in Biomarker Discovery and Development
Unfortunately, the volume of available omics data has resulted in a mathematical challenge for identifying biomarkers – something known as the “curse of dimensionality.” Essentially what this means is that when you can measure thousands or perhaps tens of thousands of potential biomarkers at the same time, you’re highly likely to identify measurements that appear to predict patient prognosis or response to treatment, but actually show up only by chance and not because of any biological connection to the disease or drug of interest.
This raises the risk of a failed clinical trial to prove the value of the hypothesized biomarker, because the biomarker doesn’t predict drug response in additional patients. In this situation it becomes incredibly important to leverage high performance algorithms to separate biomarker signal from the noise. Unfortunately, conventional machine learning and biostatistical methods struggle to separate false positive results from true biomarkers, leading to low probability that hypothesized biomarkers will validate in prospective clinical trials. Modern, advanced artificial intelligence algorithms will be needed to maximize the value of large-scale molecular profiling for biomarker identification.
How Causal Machine Learning Can Help
Causal machine learning is an advanced artificial intelligence algorithm that is very well suited to addressing the curse of dimensionality in biomarker discovery. Causal machine learning can ingest omics data to create graphical models of disease biology and drug treatment. These graphical models represent the complex molecular mechanisms in the disease and allows assessment of which molecular data predicts patient prognosis or treatment response. The graphical structure of the models is very valuable for biomarker identification, because it can distinguish potential biomarkers that are causally related to disease outcomes from those potential biomarkers where correlation is merely coincidental. As a result, causal machine learning has the powerful ability to identify biomarkers that are more likely to validate out of sample. This has a straightforward benefit in drug development: a much higher probability that biomarker discovery efforts will hold up in prospective clinical testing.
The Path Forward to Better Biomarker Driven Drug Development
Use of biomarkers is critical for translating disease biology into insights for better treatment outcomes for patients. For drug developers, identifying biomarkers as early as possible in the drug development process is key to predicting patients who might respond to a drug, enabling time and cost savings in clinical trials. Developing a better molecular understanding of disease using causal machine learning can be an important step for developing biomarkers that identify the proper treatment for each patient.
[1] Bet on Biomarkers For Better Outcomes, by Patricia Devitt Risse, MD, Life Science Leader, April 6, 2-17.
[2] https://www.pharmalive.com/annual-report-2018-state-of-the-bio-industry/
[3] https://www.ncbi.nlm.nih.gov/pubmed/23007573
[4] https://www.ncbi.nlm.nih.gov/pmc/articles/PMC4699485/
[5] https://www.ncbi.nlm.nih.gov/books/NBK338448/#IX-B
[6] https://www.accessdata.fda.gov/drugsatfda_docs/appletter/1998/trasgen092598l.pdf