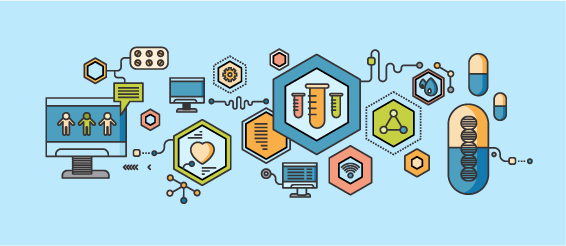
Biopharma continues to look for ways to streamline the drug development process. With costs of bringing a drug to market now reaching $2.7 billion and the timeline stretching to nearly a decade, stakeholders across the healthcare continuum are clamoring for a faster, less expensive method.
The drug research and development process is well established and has remained largely unchanged over the past few decades and for good reason – it works. The methodology biopharma has followed has given the world many effective drugs which are both improving the quality of life for patient as well as saving lives. But that doesn’t mean the process can’t be improved. The availability of powerful AI capabilities and the rapid expansion of available patient data offers a path to accelerate the process at a reduced cost (see clamoring stakeholders above).
Biopharma can now leverage AI and data to dramatically improve the earliest part of that process, drug discovery in a number of ways.
Get collaborative on data
The healthcare industry is creating enough data from EMR’s, digital imaging, natural language processing, machine learning and connected medical devices to fill three million Blu-ray disk every day1.
Unfortunately, these multiple data streams are often disconnected and biopharma can lead the way in encouraging their industry partners to break down the silos to better leverage the data they contain. Improving the data discovery process requires an ensemble of approaches that allows researchers to confidently look at the descriptors of disease to modulate pathways from the genomes to the phenotype to the molecule.
Many companies are working to obtain institutional data for their data sets, but the goal should be to create one large central data repository available to everyone. There are several examples of industry stakeholders working together to make that happen.
As part of its participation in the Accelerating Therapeutics for Opportunities in Medicine
(ATOM) partnership, GlaxoSmithKline (GSK) is helping to lead the way by providing data on nearly two million compounds and hundreds of millions of data points associated with them. Another large pharma company is in negotiation to join the group2.
The Alliance for Clinical Trials in Oncology ACTO is another consortium that is enabling data collaboration. A key component of the Alliance is allowing researchers to request data from published Alliance or legacy American College of Surgeons Oncology Group, Cancer and Leukemia Group B, or North Central Cancer Treatment Group trials.
More of these collaborations are needed to reduce the cost of data collection and ensure a steady stream of fresh data to fuel AI and machine learning engines.
Leverage the power of AI causal machine learning
There’s been a lot of discussion around the role of AI, and in healthcare in particular. It’s important to understand that there are different types of AI and which types reap the desired results. The enormous computing power of causal machine learning has the ability to change the way the industry evaluates hypotheses. Applying causal machine learning to the growing healthcare databases allows researchers to formulate and examine all hypotheses simultaneously. This enables discovery of novel insights far more quickly than having to define and test individual hypotheses one at a time – an automation of the scientific method. Machine learning enables researchers to get to causality – not simply determining the “what” but uncovering the root cause “why.”
One innovation being used in biopharma is leveraging the power of AI supercomputers to track the paths of millions of unsuccessful drugs. The theory is that many drugs fail to make it through to approval, but the information regarding cell behavior in these experiments can be beneficial. The hope is that this approach will help release a vast wealth of data and dramatically improve the search for winning drugs.
There is much that is still unknown when it comes to the drug discovery process and causal machine learning can help find these “unknown unknowns” by moving beyond curve-fitting and correlation to explore all possible hypotheses and create models of the circuitry that most likely gave rise to the data. The technology goes far beyond simply mining data for trends by discovering underlying causes and links.
Deploy resources more effectively
AI and causal machine learning will not replace doctors or researchers engaged in drug development and discovery. It will, however, automate and accelerate hypothesis generation and allow freeing up resources to spend more time on value-add activities like testing and evaluation.
Instead of having clinical experts and data scientists peel through each hypothesis one by one, they can focus on validating assumptions. Expert resources can focus their time and effort on analyzing and proving the efficacy of new discoveries.
Freeing up resources also allows subject matter experts to help frame drug development experiments and clinical trials at the critical start of the development process. This can ultimately save time in the long run by leading to more successful trials.
Leveraging the combination of AI causal machine learning and growing healthcare data bases doesn’t change the need for a rigorous scientific process. By embracing change, biopharma can streamline the research and development process, increase the chances of successful clinical trials and provide new therapies to patients more quickly and at reduced costs.
For a more in depth look at how AI and data are accelerating innovation in biopharma R&D, look for the soon to be released GNS eGuide: Transforming the Traditional Drug Discovery Paradigm.
[1] Comment: Health Networks – delivering the future of healthcare, by Jim Gerrity, Building Better Healthcare, January 24, 2014
[2] Glaxo Finds a Rich Vein to Mine with Supercomputers, by James Paton, Bloomberg, October 13, 2017.