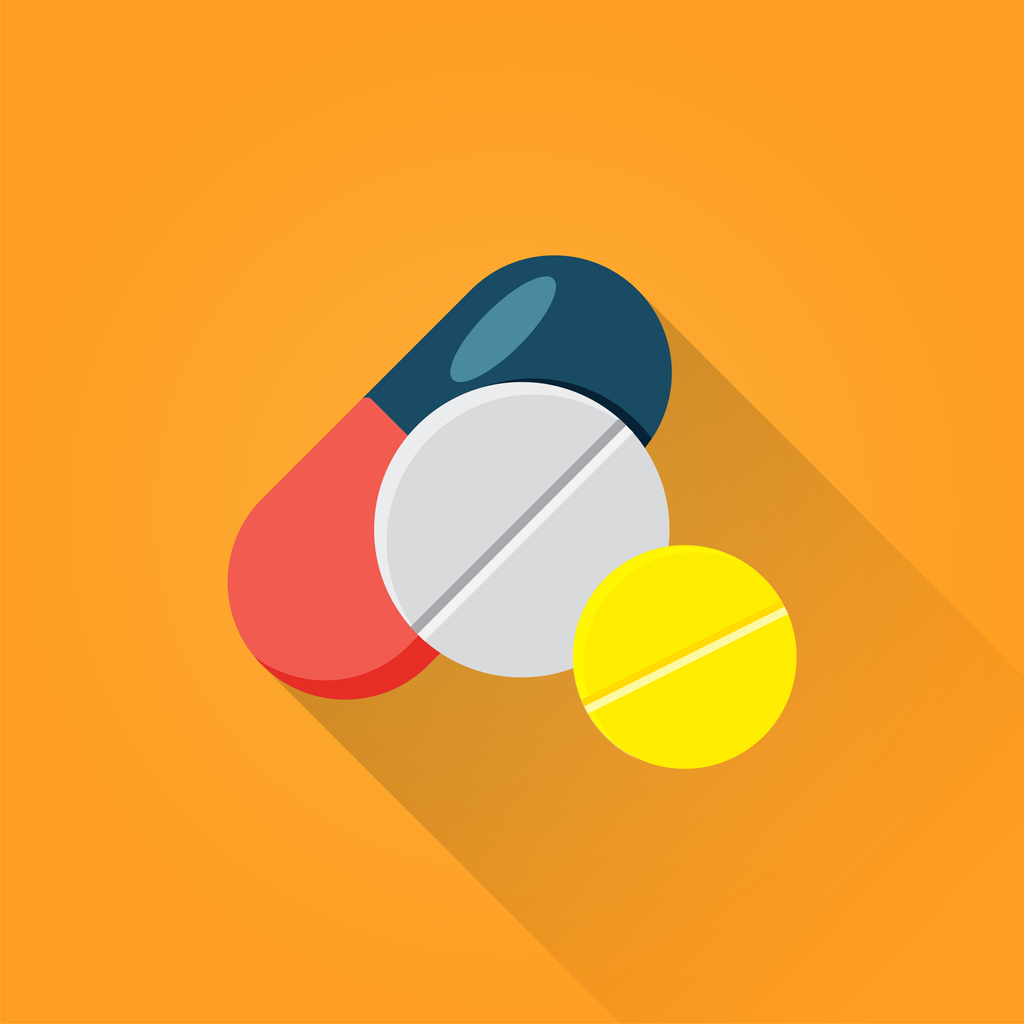
Chances are, you know of someone who suffers from multiple myeloma, non-Hodgins lymphoma or multiple-sclerosis. Despite the familiarity of those conditions, all three are considered rare-diseases by the U.S. Food and Drug Administration (FDA) because they affect less than 200,000 people in the U.S.[1]
According to estimates by the National Institute of Health, there may be as many as 7,000 rare diseases affecting approximately 25-35 million Americans.[2] So what do rare diseases have to do with AI?
Prior to the 1980s, few treatments - now known as orphan drugs - were available to treat rare conditions. That changed in 1983 with the enactment of the Orphan Drug Act which provided financial incentives to accelerate the development of products to these uncommon diseases.
The legislation provided financial incentives for the pharmaceutical industry, including a seven-year market exclusively period for drugs approved to treat orphan diseases and tax credits of up to 50 percent for research and development expenses. In addition, the law authorized the FDA to designate drugs and biologics for orphan status (the first step toward acquiring the development incentives), provide grants for clinical testing of orphan products and offer help in framing protocols for investigations.[3]
The statute had a dramatic impact on the development of drugs for rare diseases. In 1983, the FDA approved two orphan indications. That number grew to 25 by 1996 and 80 by 2017. The agency granted orphan designations to over 429 unique drugs under development in 2017. More than half of the 42 new active substances launched in the U.S. in 2017 were orphan drugs.[4]
Biopharma faces many challenges when attempting to bring orphan drugs to market including missed opportunities from patient misdiagnosis, logistical barriers to patient recruitment for clinical trials and pressure from stockholders to meet major milestones. Pricing and reimbursement, regulatory restrictions and developing a sustaining funding source are also major roadblocks to orphan drug development.[5]
Patients suffering from rare diseases have their own set of challenges, not the least of which is finding the right diagnosis. While nearly 80% of orphan diseases are genetic, the average time to diagnosis approaches five years, with patients often seeing a multitude of providers before being diagnosed. AI can help. One application of AI is to better understand the patient populations who are misdiagnosed and treated as a more common condition.[6] In this case, AI can help understand which categories of patients may benefit from screening for the genetic markers which would indicate an orphan disease. Reducing the time to diagnosis may improve patient care by enabling diagnosis and access to appropriate treatments.
Overall, the limited number of patients suffering from rare diseases is a major hurdle to developing effective therapies. While AI can help researchers leverage the growing range of biomedical information, including structured and unstructured data, to enable more effective analysis, sizable data sources to support the research are still being constructed. This approach can lead to better protocol design and patient recruitment for clinical trials and increase trial success rates.
The capacity to ingest and analyze data is an important advantage of AI and machine learning in developing treatments. In silico modeling of previous clinical trials can help analyze treatment impact, simulate clinical trials in different populations and study designs to help target which drugs should be further evaluated. Using AI technology to combine wet lab and in silico experiments enables researchers to take advantage of huge data sets to perform multiple simulations. This can lead to correlations that lead to causality and help pave the way for the development of new rare disease treatments.
AI and machine learning also enables biomarker discovery that can be used to diagnose rare disease as well as predict disease progression and individual response to drugs and therapies.
Leveraging AI to discover biomarkers accelerates the approval of drugs to treat rare conditions. In 2012, the FDA approved the use of the accelerated approval pathway for rare diseases that has since been used to fast-track drugs to treat cancer and HIV. The conventional approach to approval relies on a benefit-risk ratio that looks at tangible changes in how the patient feels. The accelerated approach enables approvals based on the drug’s impact on surrogate outcomes – biomarkers that are related to disease but not necessarily the direct outcome of the disease. Biomarkers discovered through the power of AI can help classify disease at the gene, cellular, tissue, organ, and integrated system level and lead to a clearer understanding of final outcomes.[7]
Developing treatments for rare diseases is difficult. But we are now at an inflection point where we can combine the surge of available data with powerful AI technology to ensure that all patients receive the care they deserve.
[1] Developing Products for Rare Diseases & Conditions, FDA website, accessed 6/10/19
[2] FAQs About Rare Disease, NIH, National Center for Advancing Translational Sciences web site accessed 6/10/19.
[3] The Story Behind the Orphan Drug Act, by John Swann, Ph.D., FDA Historian, FDA website, accessed 6/10/19.
[4] Orphan Drugs in the United States, IQVIA Institute for Human Data Science Report, October 17, 2018.
[5] Clinical development and access issues in rare diseases, by Global
[6] The Global Challenge of Rare Disease Diagnosis
[7] Shifting Biomarkers: The Future of Expediting and Refining Drug Delivery, by Betty Zhang, Rare Disease Review, April 30, 2018.