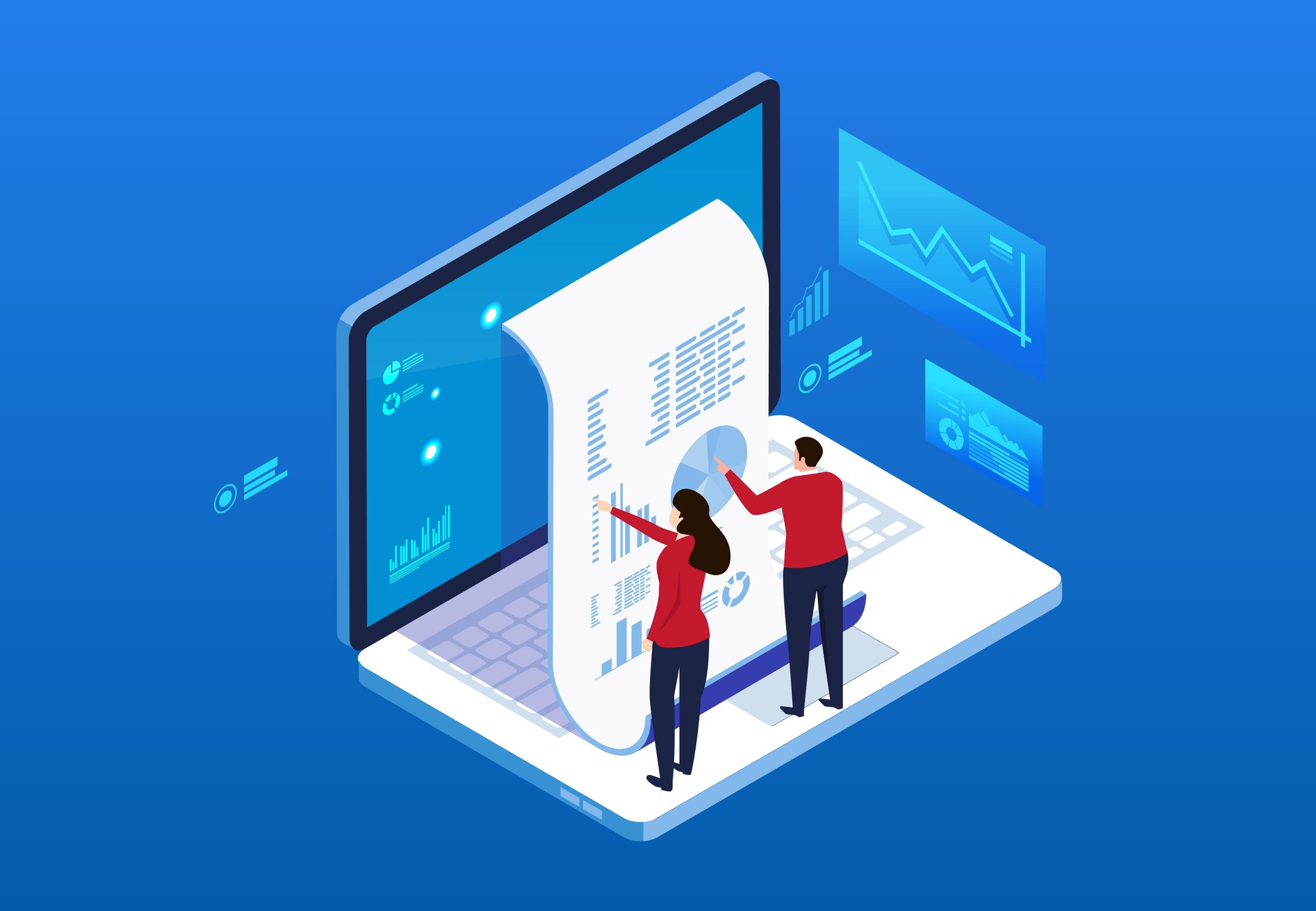
What does the future of pharma look like through the eyes of a technologist’s background? We were fortunate enough to find out at last month at our 2nd annual GNS Forum. Shahram Ebadollahi, PhD, MBA who is the Global Head of Data Science and AI at Novartis AG, joined us to share his thoughts on how to bring the promise of AI to fruition for biopharma.
Shahram started by telling the audience that not every problem is a nail requiring an AI hammer, rather defining the business problem first is crucial to making the application of AI produce valuable results. Second, a path forward for AI needs a new approach on how biopharma companies construct their teams and adoption guidelines for the powerful technology.
Beam AL, Kohane IS. Big Data and Machine Learning in Health Care. JAMA. 2018;319(13):1317–1318. doi:10.1001/jama.2017.18391
Citing from a paper by Andrew Beam, PhD and Isaac Kohane, MD, PhD, from Harvard Medical School, Dr. Ebadollahi explained the need to refocus on the man-machine mix for machine learning. The profound concern among patients and others interacting with the outputs of AI has been that the technology would replace physicians or start making medication decisions - taking the human interaction and decision making out of the equation. But such a scenario is not only a long way off, it is also unlikely given the need for a man-machine mix. The machine can optimize the processes around more mundane, operational tasks, but remains guided by humans for anything more advanced. Instead of attacking every potential problem with AI, Dr. Ebadollahi urges a different approach.
Step one: identify the problem. For example, Novartis started by conducting a survey creating a vast number of use cases which could be tackled with AI, many biopharma companies have undoubtedly done the same. The use cases can range from mechanism of action exploration, to mapping disease progression, to making interactions with healthcare providers more personalized and effective. Once gathered, use cases then need to be prioritized.
Step two: assemble the right team. A central theme at this year’s Forum was combatting silos, not solely of the data variety, but also of the human kind. Dr. Ebadollahi and others spoke of teams assembled based on subject matter expertise rather than cohesive problem-solving ability. Instead of separating the machine learning experts, biologists and market access experts, there is a case to be made for assembling diverse teams which can work together and feed off different strengths. Bringing together such a team allows a peer-level relationship among those who can together interrogate the problem and the available data sets, mapping the question at hand to the right data.
Step three: identify the right data set. The question of data availability, cleanliness and usefulness remains front and center not only at this year’s Forum but throughout nearly all conversations in the biopharma space when it comes to applying AI. We’ve discussed the problem of ‘garbage in, garbage out’ extensively on this blog and it is perhaps the single uniting factor among everyone who works in data. To unify its data assets, Novartis launched a data lake called Data42. Accounting for 20 years’ worth of clinical trial data or 2 million patient-years of data, the data lake contains information across a whole host of metrics and is accessible and quarriable by the Novartis team taking data mining to a whole new level. But the right data sets aren’t only internal to the company, Novartis has also reached across the pond to collaborate with organizations such as the Big Data Institute to review clinical and MRI data from multiple sclerosis patients to improve the company’s approach to the disease.
Step four: apply the right tools. While we talk about AI as a monolith, it is anything but. Composed of a variety of technologies and applications, the concept of AI may be nothing new but has only recently reached the scalability necessary for pharma. Following the winter of AI of the 1980s, when the distrust of AI came to a head due to a number of delivery failures, the computing power reached a threshold which made AI possible beyond a highly knowledge-intensive strategy of the 60s and 70s.
Applying the right tools means bypassing the hammer meets nail approach sometimes favored by machine learning experts and focusing instead on meeting the right business problems with the right team, and the right data, at the right time.
We were thrilled to have Dr. Ebadollahi join us for the Forum and share his expertise on how biopharma can and should embrace AI. As healthcare continues to evolve and improve it is because of leaders like Dr. Ebadollahi who balance science and technology to deliver precision medicine.
To learn more about AI in healthcare, check out our executive guide on applying AI to healthcare.