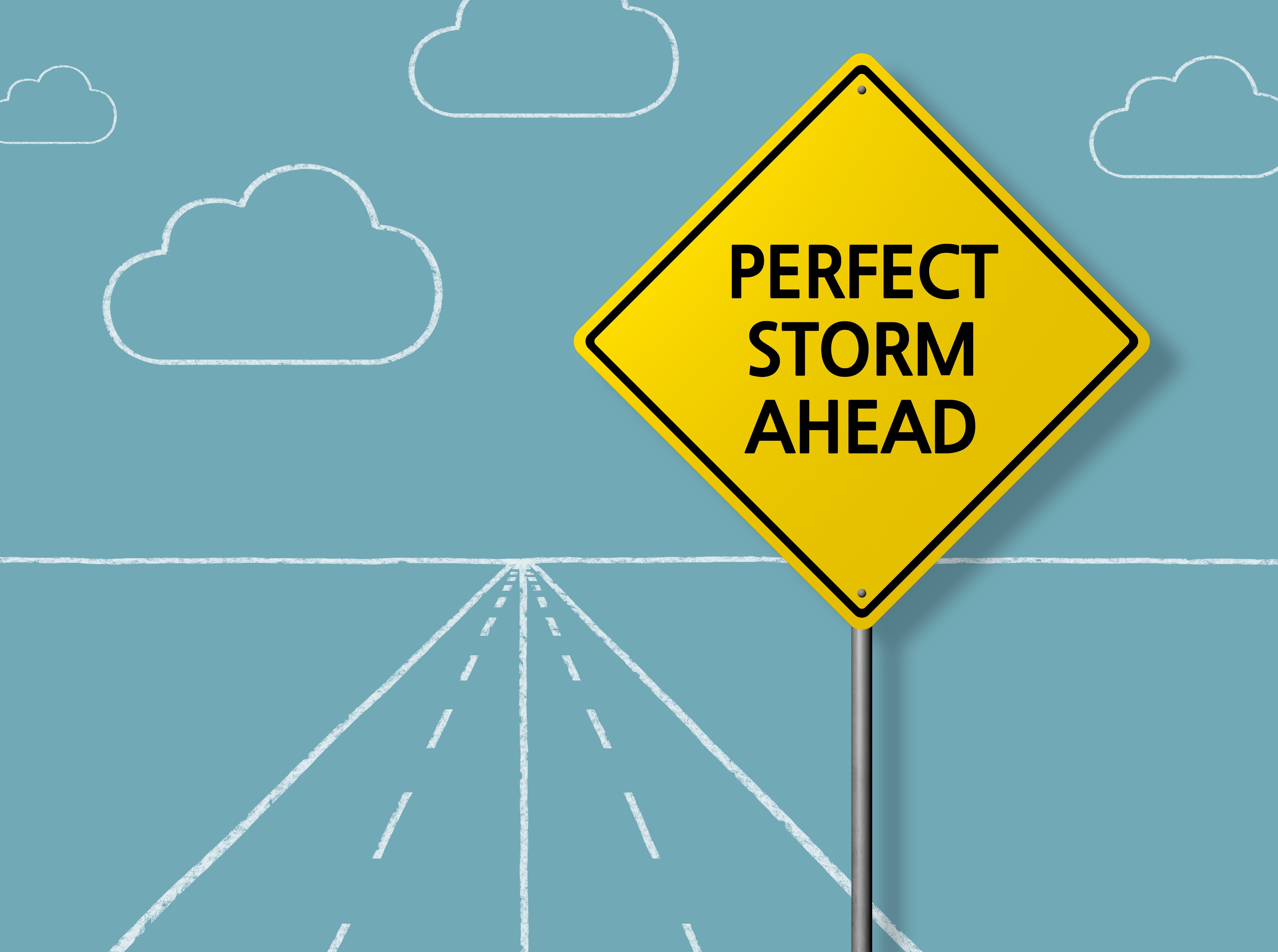
Four out of ten people with asthma don’t respond to their prescribed medicine. The same is true for half of arthritis sufferers. For cancer patients, the number is worse, with nearly eight out of ten patients failing to benefit from an FDA approved drug1.
What does this mean for healthcare? It means on average, half the treatments launched and prescribed in our three trillion dollar a year healthcare system are not effective for those they are intended to help. While these drugs have been rigorously developed, the fact is they simply don’t work for everybody.
And drugs are not the only treatment that are imprecise. Other health interventions – medical procedures, medical devices and care management programs -are just as unlikely to work on the average patient. The result is rapidly increasing healthcare costs without the desired outcomes. In the US, the cost of healthcare grew from 13.3% of GDP in 2000 to an estimated 18.2% in 2018. Annual spending on healthcare reached $3.5 trillion in 2017 and is forecasted to grow to $5.7 trillion by 20262.
Finding a way to make treatments, devices and medicines more effective has huge implications in terms of lives saved, reduced costs and improved health outcomes. Solving this problem is a fundamental challenge for pharma, health plans, providers and healthcare consumers.
The Convergence of Precision Medicine and AI
Rapid advances are occurring in both AI and precision medicine, and the convergence of the two is where the prescription for change lies.
The modern origins of precision medicine can be traced to the discovery of the double helix DNA structure by Crick and Watson in 1953. It wasn’t long after that the first-generation DNA sequencing was developed in 1977. This paved the way for the precision medicine watershed event, the human genome project from 1990 to 2003. Former president Bill Clinton hailed the completion of the project saying, “we now have the book of life.”
AI’s roots go back further than many may realize. In 1950 Alan Turing, the father of modern computing, proposed a test to determine a computer’s ability to mimic the intelligence of a human being. John McCarthy, a computer scientist from MIT, coined the term “artificial intelligence” in 1955. The 1960’s saw the introduction of Bayesian methods for AI by Ray Solomonoff and Gordon Moore proposing “Moore’s Law” predicting the doubling of computing power every two years.
A significant turning point in AI occurred in 1997 when IBM’s Big Blue Supercomputer defeated Garry Kasparov. It was the first time in history that a computer had beaten a world chess champion in a six-game match under standard time controls. Big Blue’s victory was regarded as a sign that artificial intelligence was getting closer to matching human intelligence.
While these two fields have operated independently for decades, they are now converging, resulting in several exciting recent developments in both AI and precision medicine that are making it possible to dramatically change the course of healthcare.
The first has to do with the growth of variety of healthcare data. The volume, velocity and variety of data involving everything including next gen DNA sequencing, cardiomics, traditional EHR data, clinical data and longitudinal claims data are growing at an exponential rate, and are fueling the discovery of what works for whom.
Technology is the other key ingredient to improving healthcare. Forward leaning organizations are using advanced machine learning and AI to drive the generation of insights at a scale that is commensurate with the size of the data and the incredibly complex biological and behavioral systems at play in healthcare.
The combination of vast data availability and enormous computing power is now enabling us to advance from standard reporting, statistical analysis, forecasting and extrapolation, and predictive modeling to the peak of Tom Davenport’s Data Analytics Pyramid – optimization and inference.
What’s the point of predicting the future if you can’t change it?
The ability to not only anticipate what will happen next, but to also prescribe actions that can change outcomes is where new methods of machine learning shine – specifically causal learning and simulation. Creating causal models now enables healthcare stakeholders to understand the biological circuitry and simulate many possible futures under interventions to help determine the best course of action at the individual patient level.
This convergence of AI and precision medicine gives us the capability to drive and generate insights at scale across the drug development process: from the discovery of biomarkers of response, to the discovery of new drug targets, to uncovering the right pathways in a disease system to drive better outcomes.
We now have tangible examples of what this technology can do in diseases like multiple myeloma, Parkinson’s and colorectal cancer. It’s not just about these particular discoveries though, but rather the fact that there is now a capability and technology that can drive these kinds of validated insights.
The impact of the convergence of AI and precision medicine can be significant. One forecast says using machine learning to tailor treatments and increase patient engagement can reduce our healthcare expenditures 5-9 percent3.
A Harvard Business School article says AI and machine learning can create up to $150 billion in annual savings for U. S. healthcare by 20264. The astounding thing is that these numbers actually may be underestimating the impact of the possible savings.
Watching the rapid advances that are being enabled by the coming together of AI and precision medicine has made one thing abundantly clear. When it comes to finding solutions to the complex challenges facing the healthcare industry: the time is now.
[1] The Personalized Medicine Report, 2017 – Opportunity, Challenges, and the Future, Personalize Medicine Coalition
[2] U. S. healthcare spending to climb 5.3 percent in 2018: agency, Reuters, February 14, 2018.
[3] Machine Learning and AI in Healthcare Coming Up Short, Kx Systems, December 19, 2017.
[4] 10 Promising AI Applications in Health Care, by Brian Kalis, Matt Collier, and Richard FU, May 10, 2018.