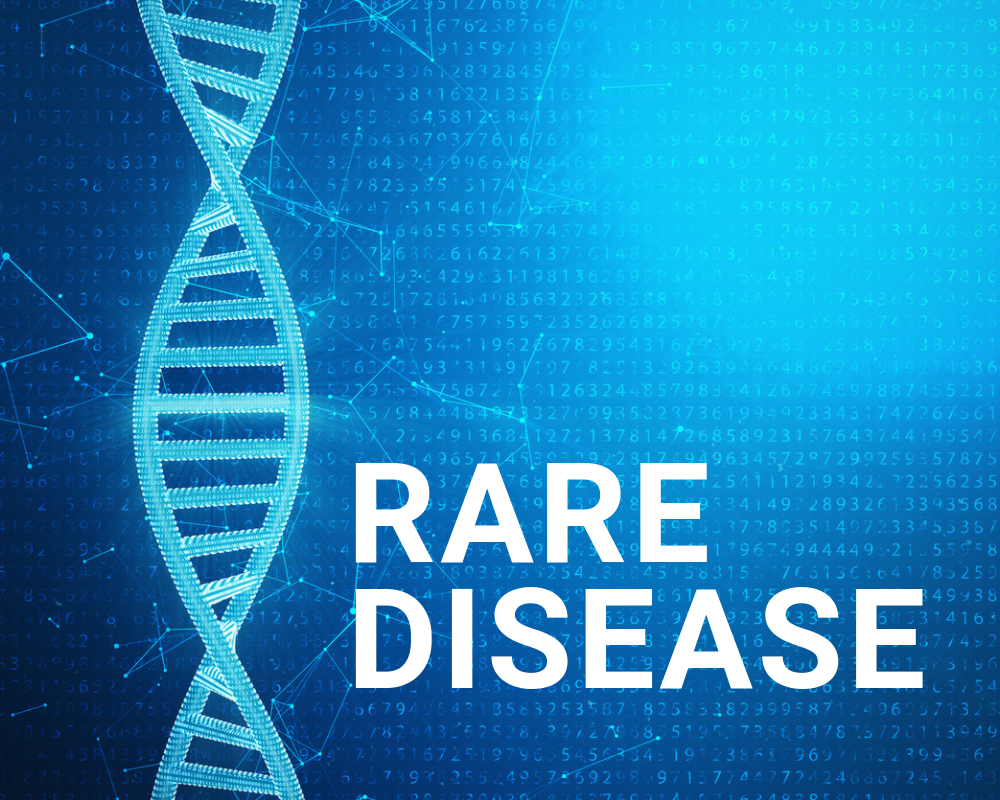
The Orphan Drug Act of 1983 defined a rare disease as one that affects less than 200,000 people. According to the NIH National Center for Advancing Translational Sciences, there are now 7,000 such diseases afflicting between 25-30 million people in the U. S. and an estimated 350 million worldwide.
Finding therapies for rare diseases not only helps those affected, but according to studies from the NIH Undiagnosed Diseases Program, new discoveries made from studying these diseases can also provide insights into more common conditions.
At Alexion, we are interested in understanding the rare disease landscape. Piecing together information on rare diseases can be difficult—there are often numerous names for the same disease and many disease subtypes. We pull together diverse data on rare diseases, including new onset information, incidence and prevalence, census and population data, as well as unstructured data from medical literature. We include phenotype data to try to assess the severity of disease and map every clinical trial, related molecules, the genes and underlying mutations. The result is a data graph that can answer questions on the genetic architecture of various rare diseases, their manifestation in specific populations, and paths to intervention.
Another emerging area we are leveraging is experimental data. Panomic, gene expressions, metabolic, proteomic, and specific disease tissue assays or iPSC samples that are derived from patients are all relatively new. Mining this type of data provides a great opportunity for artificial intelligence in general and specifically machine learning, but we need to be thoughtful in our approach.
Generating value in healthcare with AI and machine learning
Machine learning and artificial intelligence are healthcare’s new buzzwords, just like “Big Data” was five to ten years ago. There are many platform companies that are now branding themselves as AI and machine learning companies, but we in healthcare need to be careful consumers.
True artificial intelligence technologies are very powerful and can be appropriate solutions to many problems, especially in the area of rare disease study. The availability of computing power combined with mathematics can create impressive algorithms. But an in-silico-only course may not be the best approach to answer your question.
There is a great opportunity to bring together wet lab and in-silico experiments where we can leverage the huge data sets now available by using machine learning to quickly perform multiple simulations. With a high-quality data set derived from well-designed experiments, one can go beyond classic techniques that find correlative relationships to probe causality in the model system. Our internal experts consider very carefully the design of the wet lab experiments and set the parameters for what the in-silico experiments should be.
If we think broadly about data sciences, there are many more applications beyond drug discovery, whether they be in manufacturing, supply chain optimization, clinical trials, or commercial strategy. Data science can help us understand patient populations and align R&D and commercial colleagues, creating the opportunity to make true enterprise impact.
Real world application of AI
Our partnership with the Manton Center for Orphan Disease Research at Children’s Hospital in Boston is a good example of an initiative that can further the use of AI in a real-world setting.
Under the collaboration, we are sharing our 20 Rare-Disease Questions (20RDQ) platform which integrates a comprehensive landscape of rare disease definitions with clinical feature databases to enable a series of guided questions based on a patient’s medical history and record. Using AI, we can now combine rapid genome sequencing and phenotypic signatures to produce a prioritized list of suspect genetic variants of rare diseases for consideration by a diagnosing physician.
To gain novel insight into specific areas of network biology, we licensed GNS Healthcare’s causal machine platform (REFS) as part of a broader collaboration with Sema4. This combined approach yielded remarkable deconvolution of the underlying systems biology architecture under investigation.
Machine learning approaches hold great promise for addressing the challenge of quickly and accurately diagnosing rare diseases. It is collaborations such as this that combine expert clinical insight with cutting edge artificial intelligence that have the potential to make a profound difference for patients and their families.
Leveraging partnerships is key
Alexion has an internal team comprised of high caliber, very senior data scientists. Building and replicating existing technologies can be a big lift, so sometimes, in order to achieve scale, you need to enlist good partners and platforms.
When partnering, our data science teams form a critical nexus of integration. Our internal experts distill and understand the dozens of platforms out there and determine the best technologies for the challenge at hand. Then we pair our internal experts with best-in-class partners to create effective virtual teams. We look for partners that can do more than simple correlation and instead get to causation.
There are some organizational challenges. You need to have incredible internal data science talent. It used to be that you could hire five to ten scientists and teach them the tools they needed to use. But data science is so challenging now with such a huge toolbox that organizations more and more are hiring data scientists and teaching them the necessary life sciences.
Ultimately, it is a balance. You need to look for demonstrated learning agility, talent that can move between different problem domains with the ability to apply advanced data science tools to life sciences.
The ability of our data scientists to leverage AI and machine learning through innovative partnerships to access, analyze and discover causal relationships from multiple data sets from various sources has great potential to change how we treat rare diseases. Massive computational firepower combined with advanced mathematical algorithms can speed up the drug discovery and development process and ultimately make the development of drugs for these orphan diseases more efficient and feasible – enabling the industry to tackle a broader cross section of the rare-disease landscape and bring new medicines and hope to patients and their families.