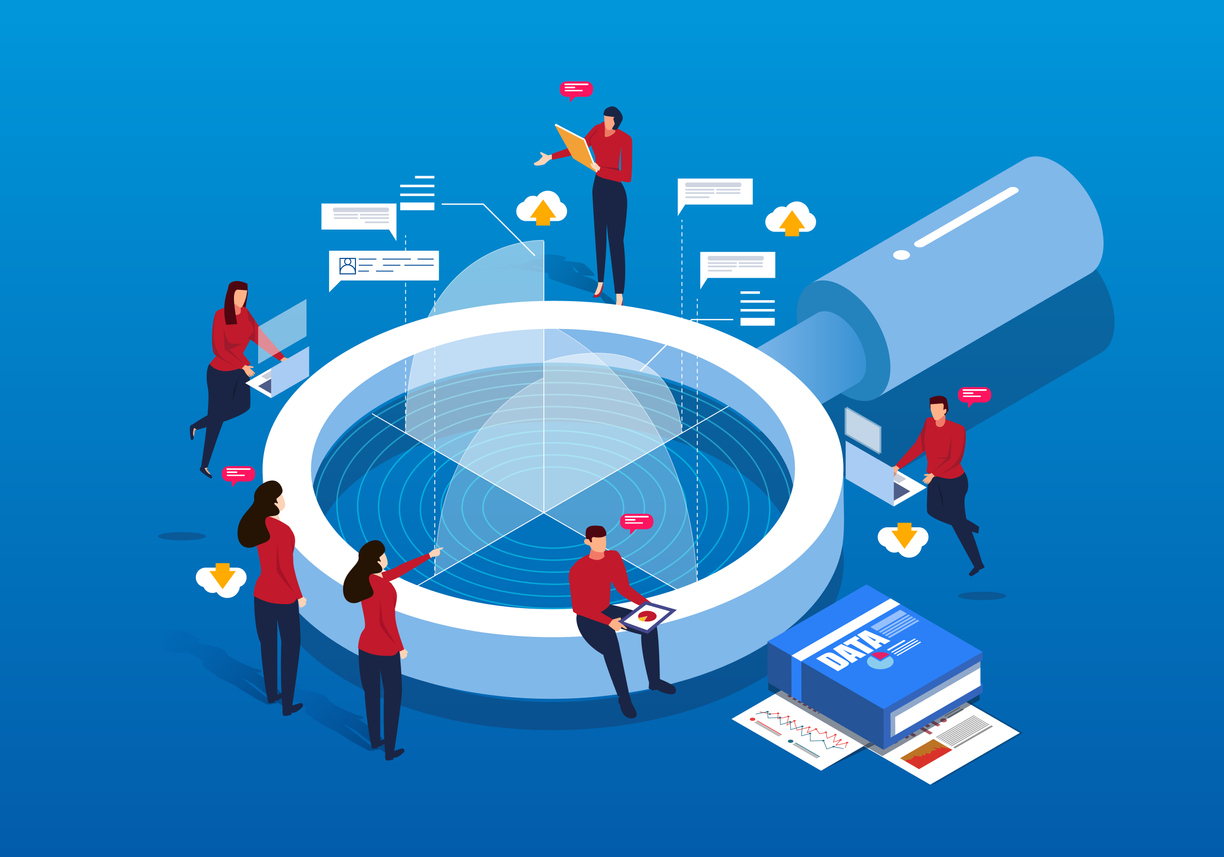
Much research and analysis in healthcare is based on testing preconceived hypotheses – looking to answer a narrow set of questions in the form of a hypothesis. Traditional statistical analyses begin with an explicit hypothesis – a specific statement of how variables interact to explain an observation or phenomenon – which is then statistically tested to confirm whether or not the data supports that position.
Artificial Intelligence (AI) is increasingly being used to carry out this type of healthcare testing from drug discovery to targeted interventions at the point of care. We have seen the impact of AI in its ability to help diagnose diseases and predict which patients are likely to suffer from illness or require intervention. But this is the beginning. There is an increasing need to study more variables and discover insights we didn't realize we didn't know to hypothesize.
The limitations of preconceived hypotheses
Traditional analytical methods assume a certain relationship between different variables. Testing a preconceived hypothesis either validates or rejects the hypothesis, but does not reveal the underlying relationship between the variable. In other words, the why.
Establishing different hypotheses can be sufficient when the number of relevant variables is small, because there is a manageable limit to the number of ways the variables can combine to explain the data. However, with the increasing amount and complexity of genomic and molecular data now available combined with the sheer number of variables to be examined, traditional techniques are not enough. With the enormous size of healthcare data, the number of possible combinations – the specific hypotheses that could explain the data – explodes exponentially. It literally becomes impossible to formulate and examine each of them. Uncovering insights with a massive data set containing many possible variables requires a different kind of AI: hypothesis-free AI, also known as causal machine learning.
Causal machine learning works by transparently discovering the cause and effect relationships within data, with no preconceived hypothesis. Rather than seeking an answer to a preconceived question, the hypotheses-free approach allows the data and relationships within it to drive the answer. It's important to understand that it doesn’t abandon hypotheses as much as it frees them from the constraints of manual formulation and examination. This approach allows researchers to examine a vast number of hypotheses simultaneously to identify the small set worth spending more time to interpret and test.
The hypothesis-free approach allows causal structures to be inferred from the data. Discovering underlying structures – whose components show how variables are connected - is key to understanding the mechanisms of disease. Models can be simulated to quickly examine a range of possible changes that allows researchers to quickly distinguish which ones are critical and which are of little value when it comes to further analysis.
Human biology is complex with much still to learn and much that is still not understood. By transforming millions of data points – clinical, genetic, genomic, lab, drug, consumer, geographic, pharmacy, mobile, proteomic data and other emerging sources - causal machine learning calculates and explains the causal relationships that drive the specific outcomes at scale.
So why is this important? Because it discovers which treatment or intervention works for which patient, which is critical to develop targeted treatments and drugs, or understanding why one drug is better for patients with a specific biomarker.
Providing a “white box” solution
Causal AI also addresses one area of major concern for researchers: the lack of visibility into the mechanisms that produce output. Causal AI and hypothesis-free testing is based on a Bayesian mathematical foundation, so the computations used to derive the outcomes is completely transparent and explainable. This “white box” solution breaks open the AI “black box” to enable researchers to see exactly how the output was reached. This increases the confidence in the conclusions and improves the chances that the results will be accepted and implemented.
Healthcare research has become too complex to rely on the traditional, limited hypotheses approach. Adopting the hypothesis-free approach to research is the most effective way to get to the root causes of disease and uncover the most effective treatments and therapies.
The problem of data bias
As effective as causal machine learning and hypothesis-free testing are, the insights discovered are only as valid as the data used to obtain them. If the populations from which the data is collected isn’t diverse, the results may be biased. For instance, if a study on heart disease uses data from a mostly white males, the results AI delivers may not be as relevant to minorities and women.[1]
The effectiveness of any analysis method rests on having robust, diverse data that represents a wide swath of the population. The All of Us research program initiated by the National Institutes of Health is striving to provide the needed data diversity by attempting to gather data from a million or more people living in the U.S. The effort aims to collect data from minority and other traditionally underrepresented groups to minimize the impact of bias in healthcare research.[2]
Despite years of research efforts and investments, our understanding of the mechanistic underpinnings of disease progression has significant room to improve.[3] But – with ballooning data availability and diversity in sources, we can move into a hypothesis-free, stage of discovery where we overcome obstacles posed by our limited knowledge of disease pathways. We now have the ability to unravel human biology, understand disease, improve drug discovery and development and target the right treatments to the right patient at the right time. And it starts with discovering insights we didn't realize we didn't know to ask.
[1] https://www.nytimes.com/2019/01/31/opinion/ai-bias-healthcare.html
[2] https://allofus.nih.gov/
[3] https://www.ncbi.nlm.nih.gov/pmc/articles/PMC2971665/