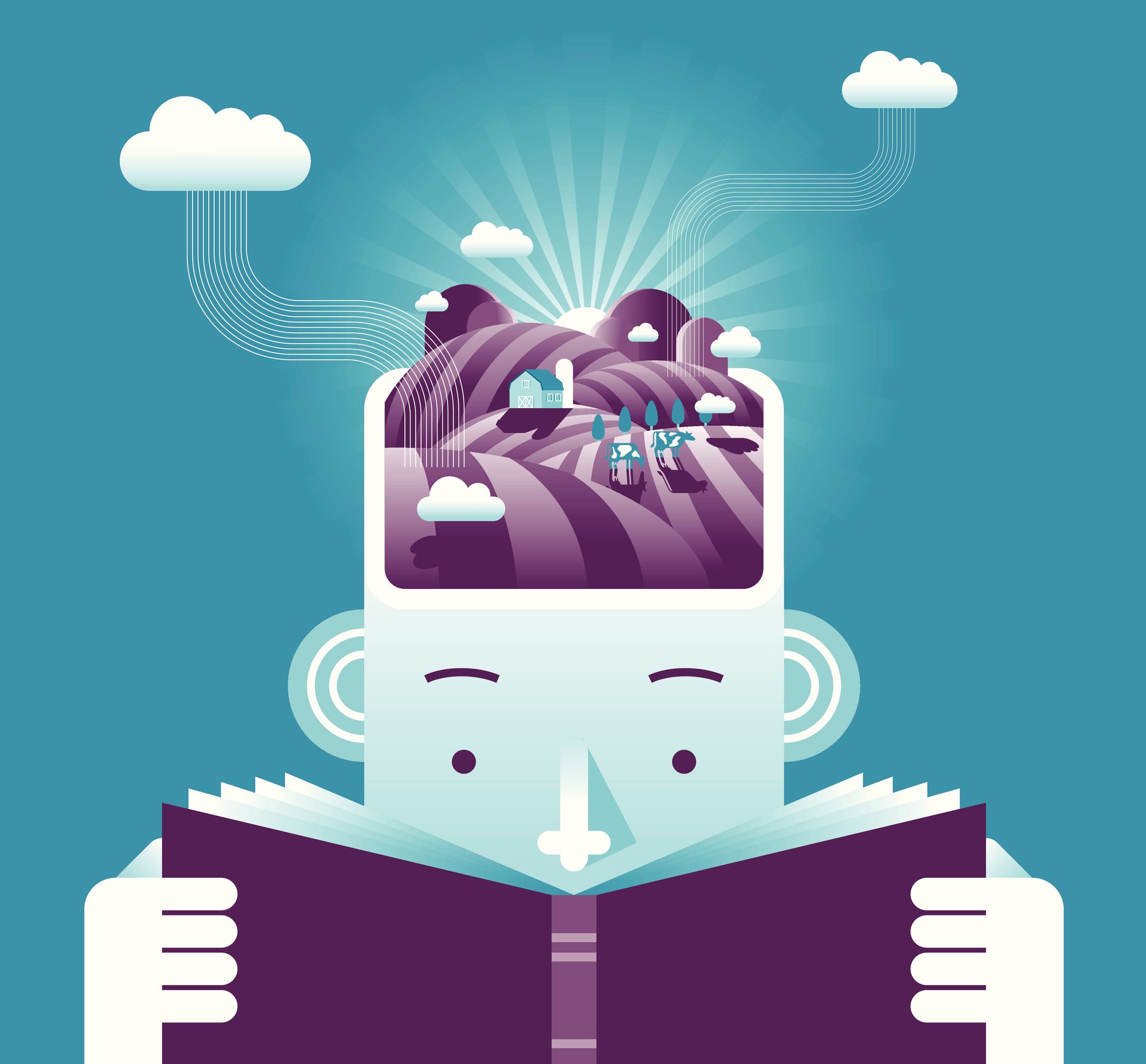
The oft-told story is that it took Thomas Edison’s 10,000 attempts to perfect the invention of the light bulb. He is famously quoted as saying of his many prior unsuccessful efforts, “I have not failed. I’ve just found 10,000 ways that won’t work.”
There is an ongoing discussion as to whether the biopharma industry should adopt a similar attitude when it comes clinical trials. A recent article in BioCentury outlined the clinical trial tug-of-war between traditional frequentists and Bayesians who have been making their way into the arena, starting first in the medical devices field. Frequentist methodology focuses on confirming or rejecting specific hypotheses from single trials, meaning that while prior information is used in the development of trial design, it is not incorporated into the analysis process for new trial data. On the other hand, ‘Bayesians’ advocate for incorporating data from previous trials to continually learn and enable analysis of multiple hypotheses.[1] This type of statistics provides a formal mathematical strategy for incorporating prior information with newly generated data during the trial.
The question is ultimately not only how to design the most optimal trial, given these techniques, but also how to establish a causal relationship between the generated data, to identify a path forward where success can be accurately measured. As the article notes, the growth in biomarker data collection paves the way for learning more from clinical trials, but only if we learn from the failures as much as we do from the successes.
To keep up with demand “drug developers are increasingly embracing methods to extract value from their clinical investments,” says GNS Healthcare Co-Founder and Chief Commercial Officer Iya Khalil, Ph.D. “Now when trials fail, no one’s learned anything.”
Failure will not be in Vain
The lack of learning from failed trials can be enormously taxing when it comes to time and money . The average cost of developing a new drug is approaching $3 billion and can take up to ten years.[2] Despite the time and expense, nine out of ten drugs fail to make it through to approval.[3] Recovering some value from these failures can help biopharma companies maximize the investment – supplying data for future trials that, even decades later, could bring a molecule back into a given portfolio. Identifying biomarkers in early trials for instance, can for instance help identify subpopulations of responders and extract value from a trial that may otherwise struggle in an all-comers population. Producing evidence earlier in the drug development process can also help drive success and differentiate products or indicate when a trial should be halted.
Causal machine learning, based on a foundation of Bayesian mathematics, transforms vast amounts of disparate data, including data from failed clinical trials, real world data, claims, laboratory, genetic, genomic and others to accelerate the discovery of what works for whom and why. Unlike traditional AI platforms, causal machine learning analyzes data sets beyond correlation, instead discovering cause and effect relationships within the data.
Causal machine learning technology gets smarter over time as it ingests additional data, revealing unforeseen relationships and novel insights. It learns by unraveling the complexity of biology, discovers biomarkers and subsets of patients who respond to specific drugs, and reveal disease pathways.
The Bayesian Advantage
“In a world where Phase II studies only measured a handful of things, you may not need a sophisticated modeling effort to understand what underlies your data,” says Iya Khalil, Ph.D. “But we no longer live in that world.”
As the debate between methodology continues, AI and causal machine learning can become key drivers of change in making trial design more adaptive. The strategy can help pharma companies remain competitive by taking innovative approaches that drive the creation of new therapies and demonstrate the value of late-stage clinical and newly marketed products.
Transparency is built into the Bayesian approach and this AI becomes a white box. While challenging to design a protocol that can respond to every potential scenario, Bayesian trials allow for learning from past failure, thereby capitalizing on previous work.
With as much time and money that is invested in clinical trials, walking away empty handed is beyond disappointing. The true cost is more palpable when one thinks about the patient lives impacted by slower, more costly access to treatment. Rewriting the status quo is a natural way forward – advancing not only the standard approaches used for trial design, but also the legal framework in which research and development are conducted. Taking a Bayesian approach and leveraging causal machine learning to incorporate previously generated data into future trials allows pharma to bypass the Edison model of 10,000 failures for ultimate success. Shedding light on drug failures paves the way for developing the right drug for the right patient faster and more efficiently.
[1] A Frequentist and a Bayesian Walk into a Trial, by Karen Tkach, BioCentury, March 22, 2019.
[2] Tufts examines $2.87bn product lifecycle cost per approved drug, by Melissa Fassbender, Outsourcing – Pharma.com, March 14, 2016.
[3] Why do clinical trials fail? Clinical Trials Arena, September 11, 2015.