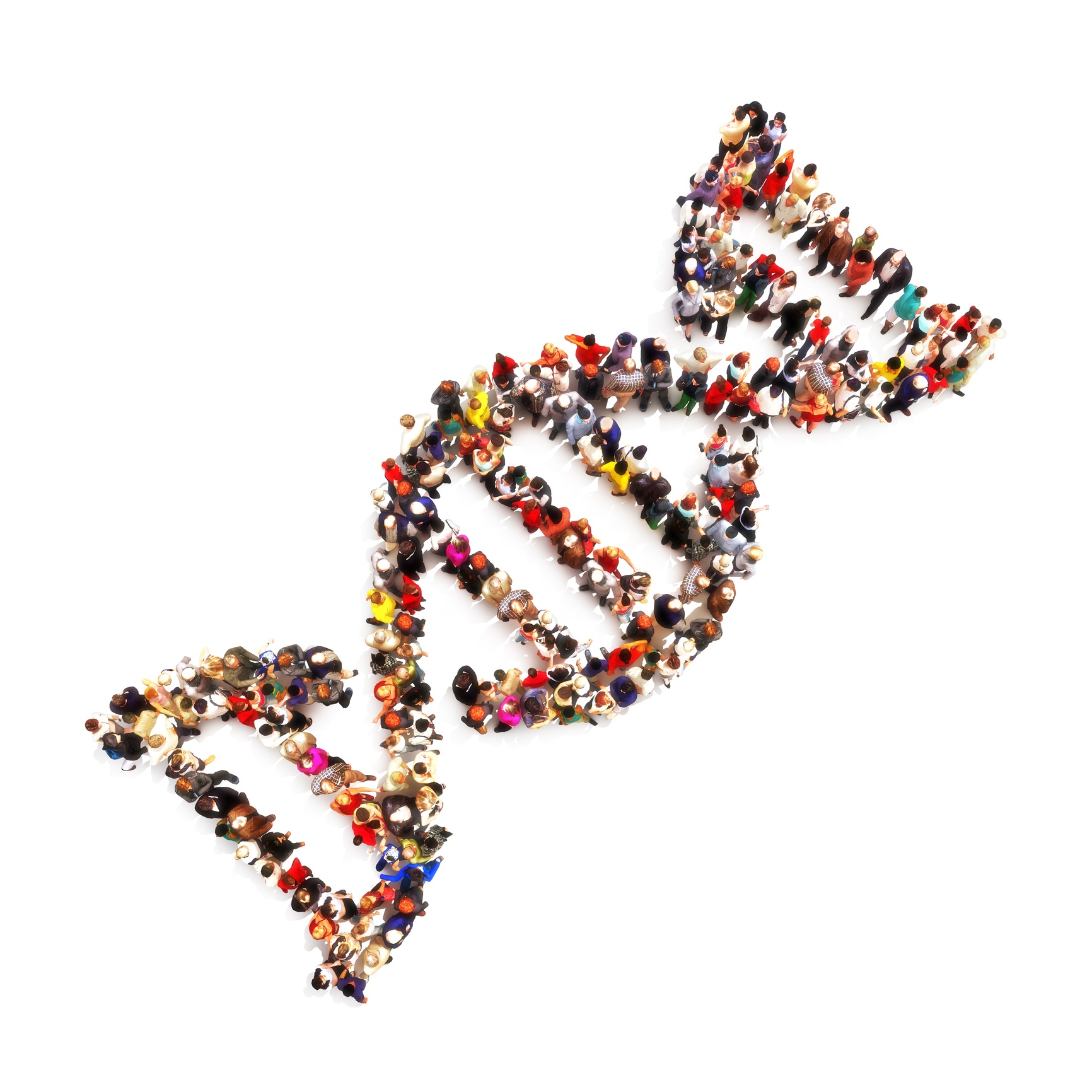
A recent study revealed that nearly half of all pipeline compounds and close to three quarters of oncology compounds are utilizing biomarker data during the drug development process. The same report indicated that investment in biomarker identification by biopharma has doubled over the past five years and is forecasted to increase over the next half decade. [1]
Biopharma’s increasing reliance on molecular data (most commonly genomic and proteomic) and the identification of specific biomarkers in the drug development process should not be surprising. Healthcare is transitioning to value-based care models and biopharma companies are being asked to demonstrate better outcomes at lower costs. To maintain financial and commercial viability in the emerging era of precision medicine, life science companies are feeling pressure to show both the efficacy of their drugs prior to FDA approval and their effectiveness to gain adoption in the marketplace.
The best way to demonstrate value is through the identification of specific groups of patients, or subpopulations, that benefit most from their therapies. One way to define these subpopulations is to identify the common biomarkers that set certain individuals apart from the whole.
The growing affordability of sequencing costs combined with the ability to measure a variety of ‘-omics’ from the smallest amount of tissue now makes it possible to identify these subpopulations in an unbiased, data-driven manner.
Machine Learning as the driver of subpopulation identification
Machine learning, a powerful form of AI, is the ability of computers to learn from data without being explicitly programmed by a human, and is a key driver that enables subpopulation discovery. Biopharma companies have succeeded in using advanced analytics to determine correlations within data, but healthcare demands causality – the need to understand the underlying mechanisms of systems.
Causal Machine Learning (CML) is providing the discovery of valuable new insights and the breakthroughs necessary to take healthcare from correlation to causality. CML accelerates the discovery process, elucidates drug mechanisms, and helps quickly identify critical subpopulations to streamline the drug development process.
Enabling biomarker identification
According to a survey from BIO, the world’s largest biotech trade association, when biopharma companies included a selection of specific biomarkers in their clinical research, regardless of stage, they saw their drug development programs’ Likelihood of Approval (LOA) rates increase by a factor of three over those that did not (25.9% to 8.4%).
Attempts to identify robust biomarkers fall flat without the use of causal machine learning. Traditional statistical methods that rely on predictive modeling fail to analyze the complex relationships in the clinical and preclinical data. Causal analysis is a necessary step in identifying biomarkers and without it, the process will likely lead to inaccurate patient identification.
A lack of a clear understanding regarding causality and the confounding relationships among key variables can mean that common statistical methods may result in mistakenly selecting a confounder rather than a true driver of the clinical outcome or treatment for further study.
CML eliminates these roadblocks, leads to effective biomarker discovery, and helps drive a successful drug development process.
Accelerates the clinical trial process
Bringing a new drug to market can take over a decade and billions of dollars, so innovations that accelerate the process provide value to biopharma and ultimately patients. CML allows researchers to explore all potential biomarkers and the wide variety of interactions among variables to select the most relevant ones for a trial. CML reduces the time it takes to identify accurate, robust, and commercially viable biomarkers. Insights that previously took months to generate can now be discovered in weeks, accelerating the path towards later stage trials.
CML also helps researchers understand the cause-and-effect relationships within initial trial data. By discovering which patients benefit from the drug in relation to the trial population as a whole, researchers can quickly and accurately refine the inclusion/exclusion criteria for later trials, helping to reach drug approval faster.
CML eliminates the problem of trying to develop a drug without a known biomarker or worse, the wrong marker. These insights help prevent trial failure, a huge setback that draws out the development timeline and adds significant costs
Enhances real-world viability
The power of CML also enhances the commercial viability of a therapy. Once the drug is made available to patients and physicians, the biopharma company can begin analyzing real-world evidence (RWE) based on its effectiveness for both primary indications and off-label use. This RWE can help identify other subpopulations of patients who will benefit from the drug and uncover potentially new indications for its use, greatly increasing the value of the drug in the marketplace.
CML unlocks the value within ever-expanding patient data sets, decreasing the time needed to bring therapies to the patients who can benefit most from them. Prioritizing the identification and targeting of biomarkers for drug development, enhances the chances of clinical trial success, allows for the delivery of value-based therapeutics, enables the optimization of a commercial strategy, and helps biopharma actualize the promise of precision medicine.
To learn more about how causal machine learning can uncover biomarkers that identify specific patient populations, check out our poster from the 2017 American Society of Hematology (ASH).
[1] Tufts Center for the Study of Drug Development, Impact Report, 2015