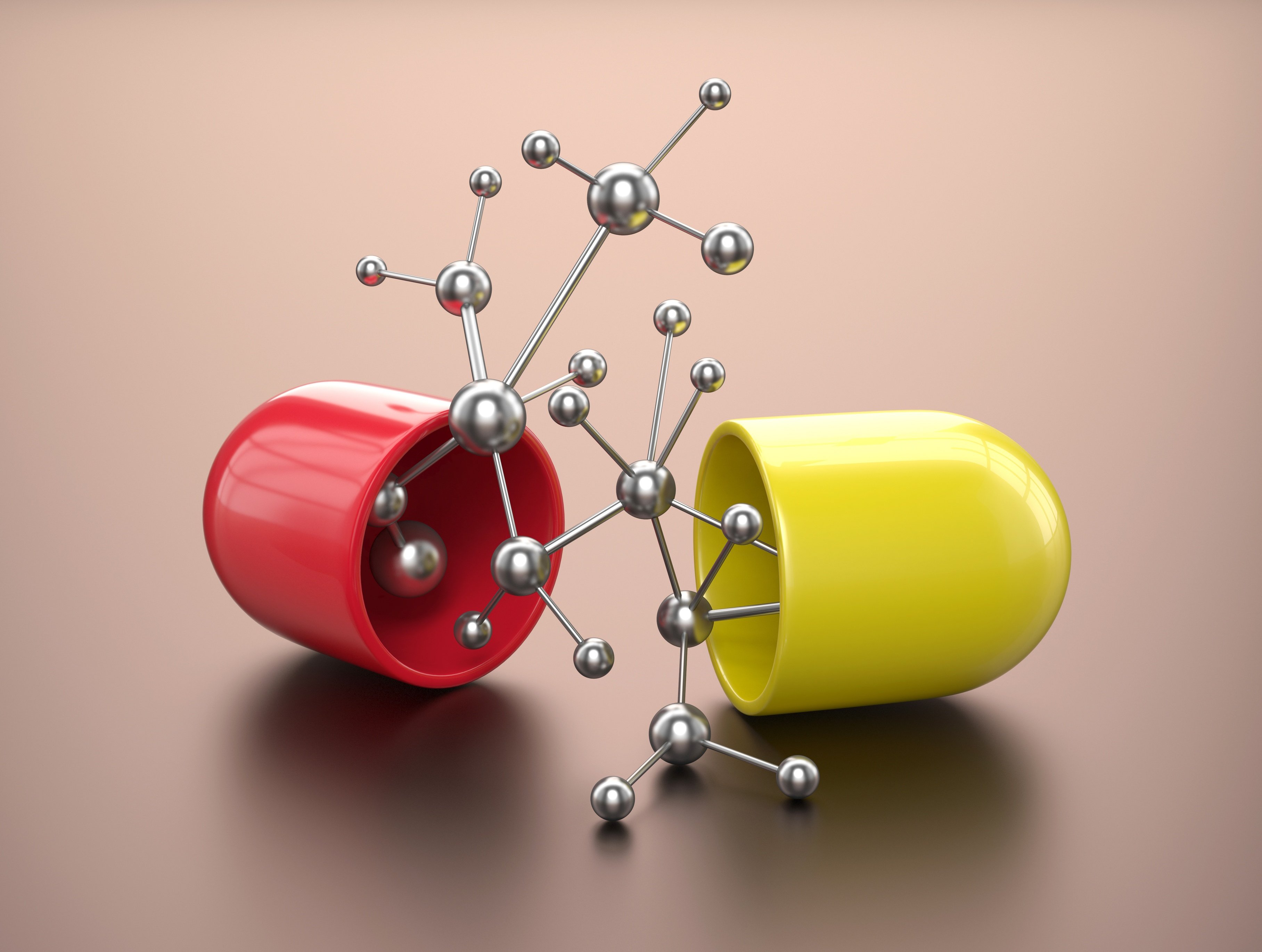
In the 1960s, physicists were trying to figure out what makes particles, such as atoms, electrons, and quarks, have mass. To answer this question, they examined existing known systems and developed complicated mathematical equations to explain and connect them, eventually coming up with something called the Higgs Field. Almost 40 years later, the Higgs Boson particle was proved to exist and is now an essential part of the particle physics model.
So what is the connection between particle physics and making improvements to healthcare? Like physics, human biology is complex and multilayered. There is much we don’t understand and can’t imagine. While tremendous progress has been made, it has taken decades and a large amount of discovery has happened thanks to technological advancements.
If physicists are able to discover hypothetical space particles and model the universe using complex mathematics, why can’t we do the same for biology and medicine?
Convergence of technology, data, and math
Thousands of terabytes of data are being generated by the healthcare industry every day. This data has the ability to fuel our understanding of preventing and treating disease, and yet data alone won’t lead to improved outcomes at lower costs.
That’s where computational biology and the use of mathematical models come into play. Creating mathematical algorithms that model biological and healthcare systems is being made possible with the use of causal machine learning (CML), a powerful form of AI. The convergence of AI and the unprecedented variety and volume of data is the driving force behind achieving the holy grail of healthcare—precision medicine.
AI’s ability to model large amounts of patient data genomic, molecular, lab results, clinical notes, EMR data – helps us understand causality. It also allows us to run simulation to predict the specific outcomes when variables like treatments or drugs are changed. This learning can be applied in many ways including helping biopharma companies improve clinical trials and working with health plans to develop appropriate intervention programs for their members.
The real promise of AI is its ability to scale, by transforming data into computer models that reveal the complex system of human disease in order to predict response to drugs and other interventions. This enables the identification and understanding of individual patient needs and care pathways – the essence of precision medicine.
There will be math
Many AI and machine learning methods used in healthcare focus on simpler problems: image identification and classification, process automation, or simple correlation. But to truly discovery the mechanics of biology and medicine, systems that are incredibly complex, the AI and machine learning technology deployed needs to built on rigorous mathematical algorithms.
Causal machine learning builds on the mathematical approaches in causality developed by Dr. Judea Pearl. He is credited with the development of Bayesian networks – a mathematical formalism for defining complex probability models and creating their principal algorithms that can be used for inference.
Instead of trying to learn a single or best model and risk overfitting, or starting with a model in mind, CML learns how trillions of small sets of variables can be connected and evaluates the ways in which they can be assembled into networks across thousands of models simultaneously. The machine then evolves these models, rapidly iterating to discover which configurations better describe a process or underlying structure that is consistent with the data.
Mathematics has unraveled so many mysteries of the universe. The mathematical foundations of causal machine learning are able to decipher biological systems as scale, enabling biopharma, health plans, and providers to deliver the right treatment to the right patient at the right time. So yes, there will be math and we are grateful for it.