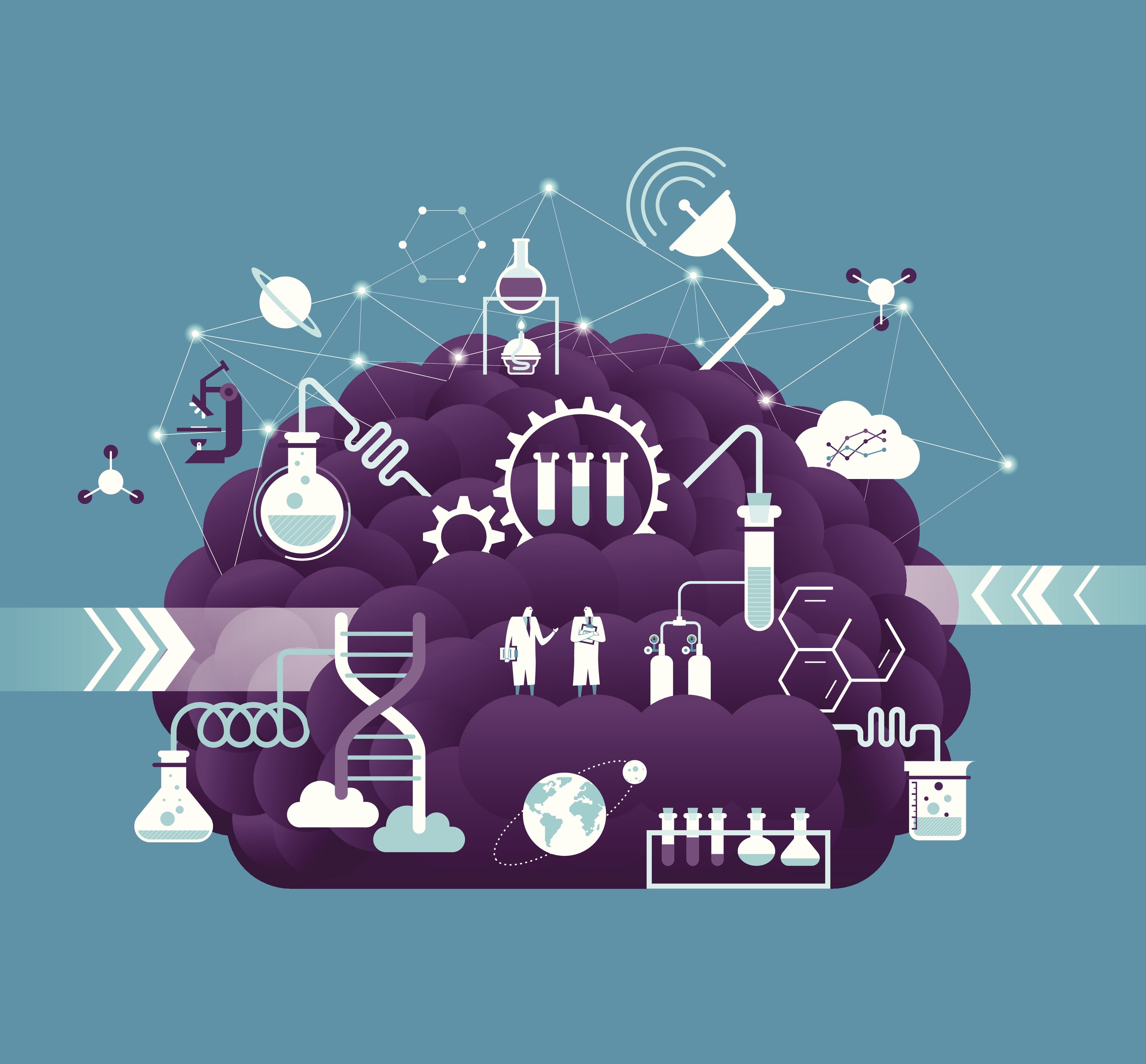
Clinical leaders at Dana-Farber Cancer Institute for Neuro-Oncology were becoming more frustrated with the lack of treatment options for patients suffering from glioblastoma (GBM). The aggressive form of brain cancer, which recently claimed the life of Senator John McCain, is one of the deadliest forms of cancer and has no known cure. Most people diagnosed with the disease die within 15 months and a meager 15 percent survive past five years¹.
Not satisfied with the slow pace of traditional randomized controlled trials studying GBM, Dr. Brian M. Alexander at Dana-Farber decided to act. A recently released Harvard Business School case study² outlines Alexander’s efforts to find a way to get faster results. He began working with a group of oncologists and statisticians to design an adaptive trial with the goal of finding new treatments for GBM faster.
An adaptive clinical trial means that the data collected during the trial, such as patient outcomes or side effects, is used to assess and modify the trial while it is still in process. The goal of this type of trial is to learn continuously from the new data created to better understand if a drug has the anticipated effect in a much more condensed timeline.
Dr. Alexander and his colleagues developed an adaptive platform trial called Adaptive Global Innovative Learning Environment for Glioblastoma (GBM AGILE) to “simultaneously and dynamically study the effects of multiple unique drugs” for the treatment of GBM. The trial is still ongoing, but efforts to innovate the way that clinical trials are conducted and accelerate the drug development process are forging ahead.
Adaptive platform trials promising alternative to RCTs
The effort surrounding GBM AGILE is just one example of a wave of new thought surrounding the clinical trial process. The recent 21st Century Cures Act and new FDA guidance has created the opportunity for biopharma companies to leverage real-world data (how a drug works when taken by patients in the real world, outside the controlled environment of clinical trials) in ways not previously possible. This patient data, combined with the growth in computing power and emergence of sophisticated AI technologies, has researchers exploring innovative ways to optimize the traditional randomized controlled clinical trial process.
So how do randomized controlled trials stack up against other strategies? While they have provided the path to approval for many needed therapies, these types of trials are limited because only one therapy is evaluated at a time. Patients are randomly assigned to one of two groups – treatment or control. Those in the treatment group receive the therapy being tested while the control group receives the accepted existing treatment or a placebo. The two groups are evaluated over time to determine the efficacy of the tested drug.
Adaptive clinical trials aim to dramatically transform this process. Adaptive trials are open-ended, as opposed to the fixed time period of randomized controlled trials, and enable researchers to incorporate new data as it becomes available, learning continuously. Treatment groups can also be added or “graduated out” as the trial progresses. Unlike randomized trials, that establish parameters at the outset of the study and maintain them throughout, adaptive models continually update the probabilities of treatment outcomes based on new data.
In silico trials - the key to adaptive trial success
With all the complexity involved in adaptive clinical trials, there is an opportunity for technology to play a significant role. Machine learning can carry out the myriad of complex calculations and incorporate new data very easily and quickly to arrive at updated conclusions about a treatment’s effectiveness. But simply relying on machine learning to do the heavy lifting when it comes to statistics misses the chance to use AI in a truly transformational way.
The real value of machine learning comes from the ability to run wholly in silico trials—or in other words, trials that are conducted completely within the confines of a computer. In order to do this effectively and in a way that is reliable and true to the scientific rigor of traditional clinical trials, you need an AI platform that helps explain the cause and effect relationships between the data, and provides transparency into what is happening and why. Causal machine learning leverages clinical trial data and real world data to create in silico trials that enable researchers to explore disease and drug mechanisms, identify subpopulations of patients through biomarker discovery, and evaluate treatment effects and responses. The way these in silico trials are built allows for a greater number and wider variety of experiments to be conducted, including head-to-head comparison studies, how a drug will perform in an out-of-sample patient cohort, and expand to possible new drug indications.
By adopting in silico trials, biopharma has the ability to learn continuously from new patient data and accelerate the clinical trial process while reducing the total cost of the trial.
Glioblastoma is just one disease that is taking people like John McCain too soon. There are hundreds of diseases that need better treatments delivered to patients faster. We now have the tools to better understand these diseases and more rapidly develop drugs, and it’s in all of our best interest to use them.
[1] Survival Rates for Selected Brain and Spinal Cord Tumors, American Cancer Society, November 7, 2017
[2] Adaptive Platform Trials: The Clinical Trial of the Future? By Ariel D. Stern and Sarah Mehta, Harvard Business School case study, January 18, 2018