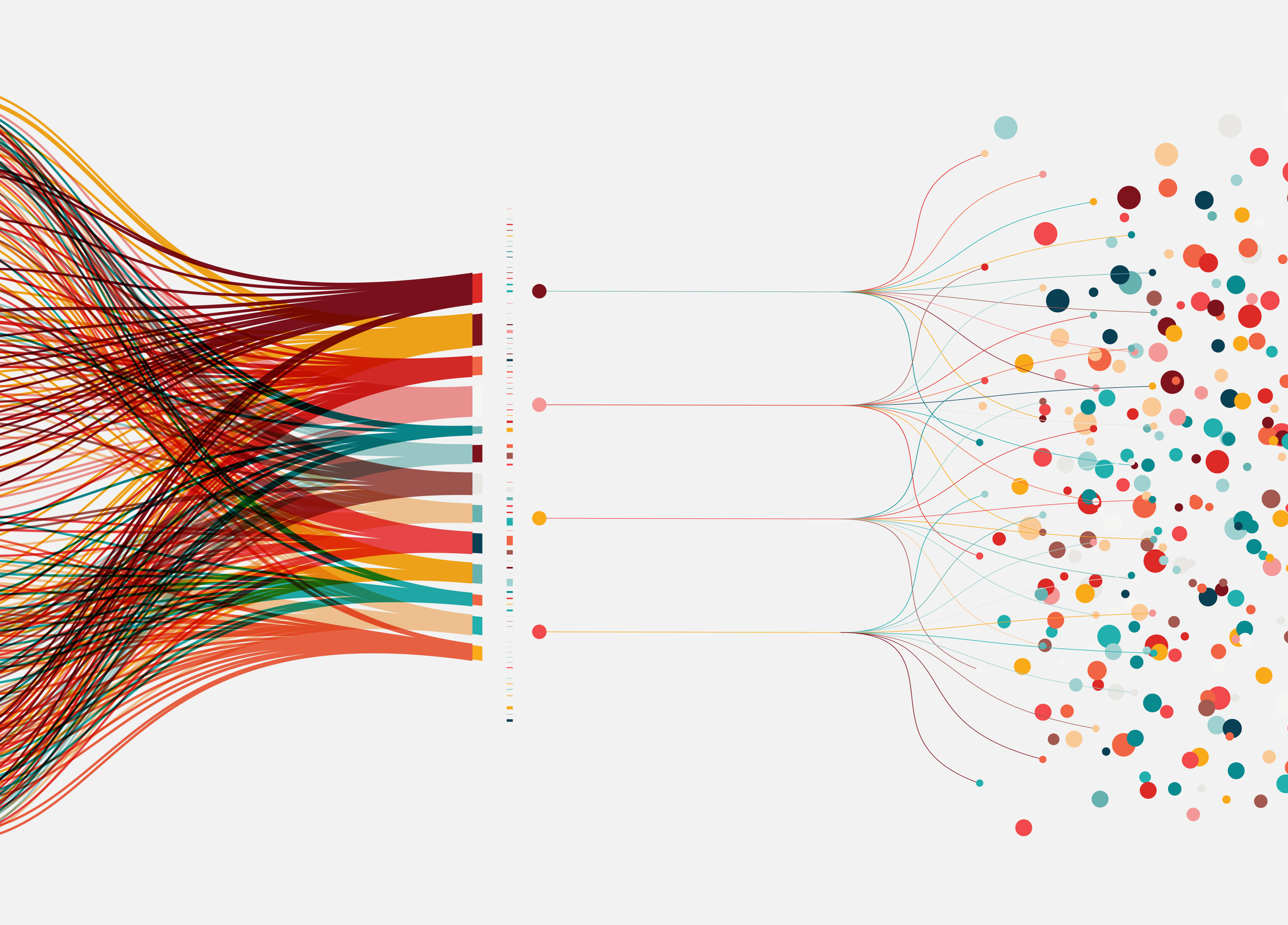
Albert Einstein is quoted as saying, "in the middle of difficulty lies opportunity." With the surge of healthcare data we have a great opportunity healthcare in a way not seen before. But, it is well known that using flawed data produces unreliable outputs. Given the breadth and depth of data that grows daily and the power of artificial intelligence (AI) – how do we leverage both to impact how drugs are discovered and developed?
A keynote panel presentation, “AI in Practice,” presented at the recent BIO-IT World Congress & Expo in Boston, examined how AI is currently impacting drug discovery and biopharma more broadly. The discussion, as reported in an article in BIO-IT World, was wide-ranging but returned to a central theme: success relies heavily on the data.[1]
The panelists focused on several key areas which lay out a clear explanation of where resources ought to be allocated to pave the way for future success.
Data Availability
Iya Khalil, Chief Commercial Officer and Co-Founder of GNS, noted that the quality and quantity of data has improved, helping to make AI and machine learning (ML) less cost-prohibitive. She stressed that finding big datasets that can virtually represent patients is key to being able to run simulations that predict optimal treatments. The level of quality required to power AI algorithms depends on the use case, Khalil said.
Khalil also mentioned the importance of leveraging open-access databases, such as UK Biobank, which researchers can use to run their datasets through to make predictions on smaller populations. Mariana Nacht, Chief Scientific Officer at Vivid Biosciences, stressed that producing some training datasets in-house makes sense as another way to utilize data.
Data Access
Susie Stephens, Senior Director, Oncology & Vaccine R&D Information Technology, Pfizer, said that collecting quality data is not enough. It needs to be stored where it can easily be reused. Pfizer, and increasingly more biopharma companies, use data lakes to make its data more accessible.
Pfizer is also talking internally about the principles of FAIR data – Findable, Accessible, Interoperable, and Reusable. Stephens notes that they “work hard to make sure data is managed robustly and is available to mine.”
Anne Carpenter, Senior Director of the Imaging Platform, Broad Institute of Harvard and MIT, noted that “privacy-preserving” data sharing was an exciting development. Khalil added that learning from all trials which can be accomplished by aggregating study data in more than just one organization, is “the dream.” With the increased drive toward collaboration among competitor pharma companies, the promise of data sharing may be on the horizon.
The panel noted that achieving data access also presents “ethical hurdles” that organizations must address. Khalil noted that it is crucial to “put patients first” and be aware of their privacy needs making sure they retain their decision-making autonomy over treatment decisions. Nacht pointed out that if bio-samples are de-identified with no link back to metadata, more people would be willing to participate in clinical studies which would contribute to greater learning.
Data Guidelines
Nacht stressed that there is a need for a consistent set of metrics around data acquisition and use.
Khalil said that guarding against bias requires “strict guidelines around out-of-sample (model selection period) and in-sample (forecast evaluation period) performance. Nacht added that algorithms are initially biased since they are trained on existing data, but that grow less so as new data is added and iterated. Stephens said that avoiding bias is another solid reason for involving subject matter experts in AI projects.
Data generation and use guidelines have also made it to the desk of the FDA, with recently released position papers on data value and governance seeking to formalize the process by which real world evidence is generated.
Data Use
As the use of AI algorithms expands, the training of the individuals who will be leveraging the growing databases needs to evolve. Khalil points out that a new generation of scientists is being trained simultaneously in biology and healthcare. Nacht said cross training will be critical and that even though experts all have their “own turf,” they need to start communicating more with each other. Stephens went on to say that expertise is needed to curate the metadata and that Pfizer uses a metadata capture tool to help automate the process.
The panel concluded that AI can clearly make a difference in the world of drug development but the vehicle for improved adoption remains the same as its always been: data, data, data.
[1] AI in Real-World Drug Discovery: The Experts Weigh In, by Deborah Borfitz, BIO-IT World, May 8, 2019