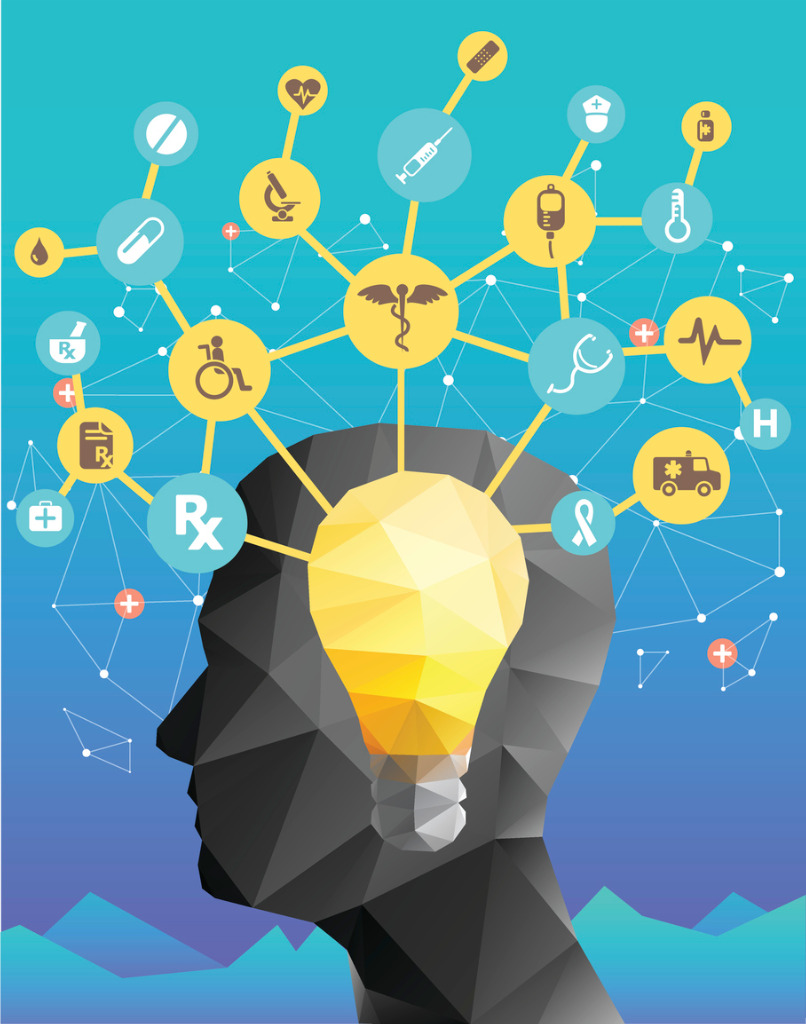
Abraham Maslow famously said, “If all you have is a hammer, everything looks like a nail.”
With all the buzz surrounding artificial intelligence (AI), it’s tempting to view it as an all-encompassing tool that can be employed to solve healthcare’s myriad of problems. In fact, there are a number of debates taking place over what AI and machine learning (ML) can and can’t do. The reality is that there are a range of AI technologies that solve very different types of problems. There are distinct use cases for each type of AI, from advanced analytics to deep learning to causal AI. But as we begin to address healthcare’s most complex questions, we need to understand which solutions - black box or white box solutions – provide the right answers.
Black box AI and deep learning
There are many types of machine learning, but the one that has generated the most conversation is deep learning. Deep learning is a type of machine learning where neural networks learn from data and classify patterns. Over time neural networks begin recognize these patterns and in effect grow smarter.
Deep learning is one of the most discussed forms of AI because it has proven to be extremely effective for several healthcare applications. Image recognition is probably the area of largest impact as it is used to diagnose early disease by detecting tumors and other anomalies often invisible to the naked human eye.
Deep learning and neural nets are helping us to learn patterns within the data. Algorithms are created that make predictions, but these predictions are created in a “black box” meaning the underlying explanation or “the why” is unknown. That doesn’t make deep learning any less effective for the problems it is trying to solve. When used to recognize patterns and make correlations deep learning is a very effective solution.
However, the central limitation of deep learning is its inability to learn the mechanism by which something happens. This is particularly problematic in healthcare. Without learning the underlying biological mechanisms, it’s impossible to identify when to best intervene to impact patient health, to understand when one drug is better than another for a particular individual, or how a disease progresses. These questions are critical when trying to cure disease or develop new therapies.
How white box AI impacts healthcare
So what does white box AI mean exactly? White box AI is a category of AI that is transparent and explainable. Causal machine learning or causal AI is a powerful form of white box AI that uses data to learn the underlying biological mechanisms and can fully explain the results.
Unlike in correlation and predictive models, the mathematics of causal inference and reasoning is able to test – in parallel- a vast number of hypotheses to determine cause and effect relationships. It helps us understand why people are getting sick, what’s driving their disease, how to identify new treatments and how to apply the proper treatment to change it.
A good example of black box versus white box AI centers on lung cancer. For years researchers struggled to establish a link between smoking and lung cancer. They knew there was a strong correlation but what other factors contributed? Perhaps improved healthcare was better able to diagnose lung cancer. Or maybe the influx of industrial pollution caused the increase. Or perhaps it was caused by a genetic disposition. Keep in mind that correlation is a measurement of how closely related two things are but doesn’t establish the cause. It took decades and following hundreds of thousands of patients to prove that smoking caused lung cancer.
Causal AI is able to accelerate these types of discoveries significantly. In The Book of Why, Dr. Judea Pearl leveraged the theory of causal inference and used causal machine learning to show how smoking causes cancer. Using Bayesian mathematics, Dr. Pearle developed a causal calculus made up of powerful algebraic rules and used them to make inferences about causal relationships. Leveraging the power of white box AI, Dr. Pearl was able look at the many possible models surrounding the causes of lung cancer. Through manipulation, he was able to remove other possible causes until smoking was the only association that persisted – thus proving causality. The white box solution had arrived.
Using the right tool for the right problem
Deep learning has accomplished many amazing things and has been instrumental in improving various aspects of healthcare. Automating administrative processes, streamlining outreach to patients, natural language processing and increasing medication adherence have all been greatly aided by this form of AI.
But as we move towards personalized medicine -getting the right treatments to the right patients at the right time – we need the ability to identify and explain the cause and effect relationships underlying the biology of medicine, which requires white box AI.
Understanding why some patients progress faster in a specific disease, why a certain gene mutation means a treatment won’t work or why certain patients respond to one therapy versus another, is a very different problem than identifying patterns in a diagnostic test. White box AI is the next phase in the AI revolution. It provides us the opportunity to learn cause and effect relationships at scale, which is what will allow us to truly cure disease and heal healthcare.