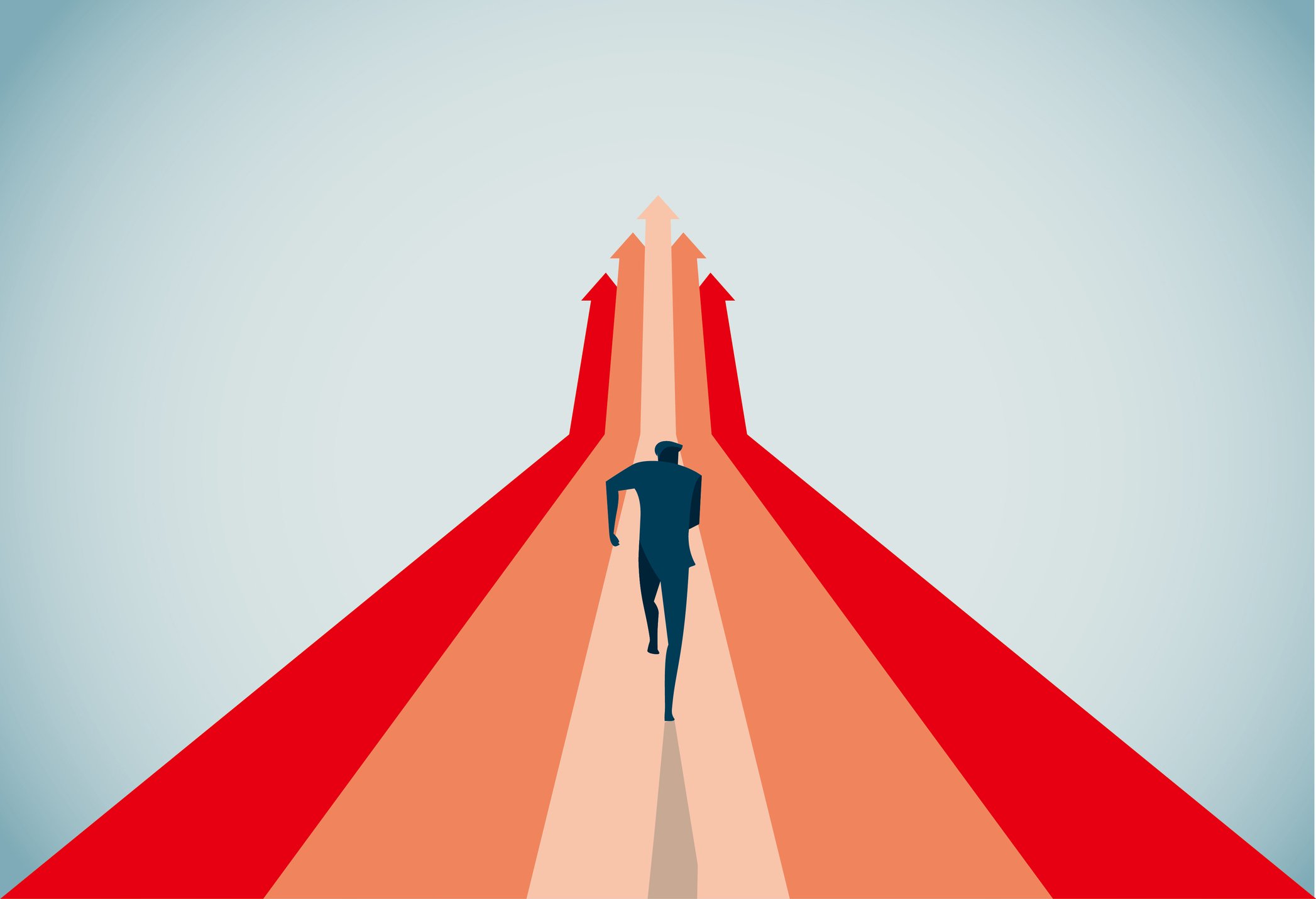
In 1896, the marathon returned to popularity as a competition in the Summer Olympic Games in Athens. The Olympic Games turned out to be inspiring to visiting Boston officials who returned home ready to challenge the locals and establish what would become one of the world’s top marathons.[1] Next Monday, April 15th, 30,000 runners will hit the road in what will be the 123rd Boston Marathon.
Across the Charles River and throughout the U.S. another race is being run, the race to bring artificial intelligence (AI) to the world of healthcare. So what does a long running (pun intended) marathon and a powerful technology have in common? As it turns out, a few key things.
Growing smarter over time
Both marathon runners and AI technology continue to grow smarter over time. Changes in running style, equipment, training methods, race analytics and technology have dramatically improved marathon performance over the past century, shortening race time and introducing spectators to a marathon lexicon that seems to grow each year.
AI also grows smarter over time. Healthcare data continues to grow and feed AI models, and with more and better data our insights and discoveries improve. Like runners, who can spend months to years preparing their bodies for the task of completing 26.2 miles, those using AI to extract insights from biology have to start by ‘preparing the data’. Runner Claire Maldarelli says “…how fast you can complete the distance in, depends on a multitude of factors including weight, sex, genetics (to a certain extend), and the energy efficiency of our form.”[2] So too does the efficacy of an AI model depend on the strength and value of the data inputs. We’ve gotten better at honing the necessary structures and preparing our data overtime, but just like runners, the more we practice the stronger we will be.
Transparency
In the past, running the marathon was a lonely pursuit. Spectators could pick a spot to watch the race but could only catch a glimpse of a runner as they passed that point. Those at the finish line were able to watch the end of the race only. They could see the result – the runner’s finish time – but had no way of knowing how the runner fared over the 26.2 -mile course.
Today, technology makes it possible to track favorite runners along the entire course – a new kind of transparency. In fact, you can receive alerts every five kilometers to check in on runners progress. The transparency allows you to understand the runner’s race and how they fare mile by mile.
Transparency in healthcare is crucial. And while not all forms of AI provide what most call a “white box” view, causal AI does. It breaks open the black box and uses data to learn the underlying biological mechanisms and fully explain the results it produces. Based on the mathematics of causal inference and reasoning, vast number of hypotheses can be tested to determine cause and effect to provide further understanding in a fully transparent process. Because unless you understand what is causing a disease or a response to a treatment, it’s near impossible to intervene successfully.
Speed
Marathon runners have been improving their speed consistently over the years. In the very first Boston Marathon in 1897, John McDermott won with a time of 2:55:10. Last year, Yuki Kawauchi won it in 2:15:58 a nearly 25 percent improvement. And the all time record is held by Geoffrey Mutai of Kenya in 2011, with a time of 2:03:02. That’s nearly an hour faster than the first winner. (For those of you who are interested, the average finishing time for all runners in last year’s race was 3:21:48).
AI too has improved its speed over time, generating insights and knowledge at a rate that is propelling discovery forward. Advances in computing power has also increased significantly, enabling AI to process faster. Once AI models are built and validated, hundreds of thousands of simulations can now be run to discover novel insights at rapid speed. We can now evaluate interactions between variables, assemble neural network models, rapidly iterate through trillions of possible configurations to make discoveries in disease and drug mechanisms. And it continues to get faster every day.
Chances are you read this blog title and thought, huh? Boston Marathon and AI, how can there possibly be parallels. But here they are - continuous learning, transparency, and speed. These three key ingredients help runners cross the finish line faster and are crucial elements of AI. These three key factors are helping runners cross the finish line and driving precision medicine.
Good luck to all those brave runners hitting the course on Monday - we are cheering for you! And to the scientists who are leading the way to improve healthcare, we offer our sincere gratitude.
[1] https://www.history.com/this-day-in-history/first-boston-marathon-run
[2] https://www.popsci.com/tips-first-marathon-science-tech#page-2