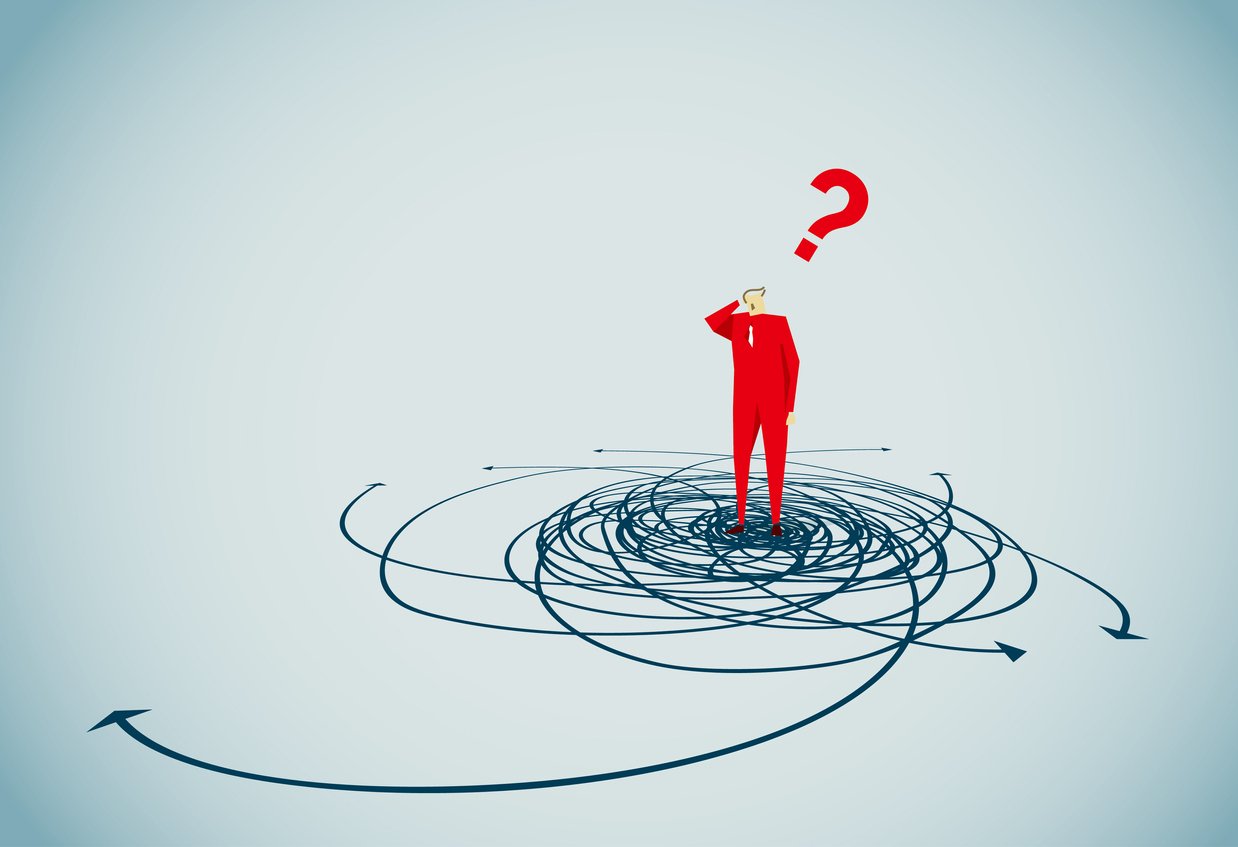
National Chocolate Day: Ten Convincing Reasons You Should Eat More of the Stuff, The Telegraph, October 2016
No, Dark Chocolate Is Not a Health Food, Healthline, December 2018
New Harvard study finds a glass of wine every day can have huge health benefits, Fox6 Milwaukee, December 2018
No Amount of Alcohol Is Good for Your Health, Global Study Says, NPR, August 2018
Why do contradictory headlines like these continually pop up, highlighting the uncertain nature of scientific studies and raising confusion as to what is or isn’t good for us? Blame it on the relative ease and dependence on the p-value.
The p-value (or probability value) is the probability that a given measurement could have come from an alternative (null) hypothesis to that which is generated by your statistical model. The p-value is a number between 0 and 1. The closer to 0, the more likely it is that the alternative null hypothesis is not true – removing the possibility that the null hypothesis is still in play. On the other hand, the closer to 1 the more likely that the result could have come from the alternative hypothesis. Often times the p-value is taken to dictate the statistical significance of the studied hypothesis, an erroneous conclusion, as the p-value dictates information only about the null hypothesis.
But should the concept of “statistically significant” continue to be the absolute measure of how we view the world? A growing number of scientists and statisticians are pushing back on the idea of the p-value and instead are accepting that uncertainty is a normal component of any study. A recent article in NPR describes the groundswell of opposition to the arbitrary p-value developing in the scientific community. [1]
Questioning “statistical significance”
Scientists are grappling with statistical variations in studies of all kinds and some are now going so far as to advocate scrapping the entire concept of “statistical significance” and embracing the ambiguity inherent in the research process.
Wariness around the concepts of “statistical significance” and p-values is not new. In 2016, the American Statistical Association (ASA) released a statement providing guidance to improve “the conduct and interpretation of quantitative science” where ASA executive director Ron Wasserstein stated that the p-value was never intended to be a substitute for scientific reasoning.[2] He stressed that well-reasoned statistical arguments contain much more that the value of a single number and whether that number exceeds an arbitrary threshold.
The ASA release outlined six principles for researchers to consider including:
- p-values do not measure probability that the studied hypothesis is true
- Scientific conclusions should not be based only on whether a p-value passes a specific threshold
- By itself, a p-value does not provide a good measure of evidence regarding a model or hypothesis.
The Bayesian Way of Thinking
Bayesian presents another way of thinking. Rather than comparing two models, a hypothesis of interest and a null counterpart, with parameters resulting from the data, the Bayesian approach allows for a more robust and clean comparison of the models in their entirety, uncertainty and all.
The advantage of Bayesian methods is you can keep the uncertainty in the data generated by your experiment, or trial. Rather than set a specific parameter for the model, Bayesians keep the uncertainty in, and compare the two hypotheses for all possible parameter values. While you could, in theory, have a Bayesian model comparison and a p-value calculation agree with each other, if you had large enough data sets and rather good models to work with, the likelihood of such simple problems and solutions crossing our desks is low.
Proponents of Bayesian methods say p-values don’t reflect the probability of a sample error in future experiments, the reproducibility of the data, the truth of the hypothesis or the practical significance of the results. Bayesian statistics remove the limits inherently imposed by estimating for a set of parameters, rather allowing all of the available data to speak. Ultimately, results from Bayesian methods tend to be more robust and direct than those generated using the common “p-value/statistical significance approach.”[3]
There are very few certainties in the world. Scientists and researchers are quickly coming to believe that unexpected vagaries prevent big questions from being proven true or false even through a rigid scientific formula. To promote true, productive scientific discussion that ultimately leads to the discovery of novel insights, research needs to consider a broader set of tools that do not falter in the face of uncertainty.
[1] Statisticians’ Call to Arms: Reject Significance and Embrace Uncertainty, by Richard Harris, March 20, 2019, Shots Health News from NPR
[2] American Statistical Association Releases Statement on Statistical Significance and p-values, ASA News, March 7, 2016.
[3] Bewitched, Bothered, Bewildered by the p Value? The Bayesian Alternative and Evidence-Based Practice in Communication Sciences and Disorders, by David L. Maxwell, PhD, and Eiki Satake, PhD, American Speech-Language-Hearing Association, March 2017