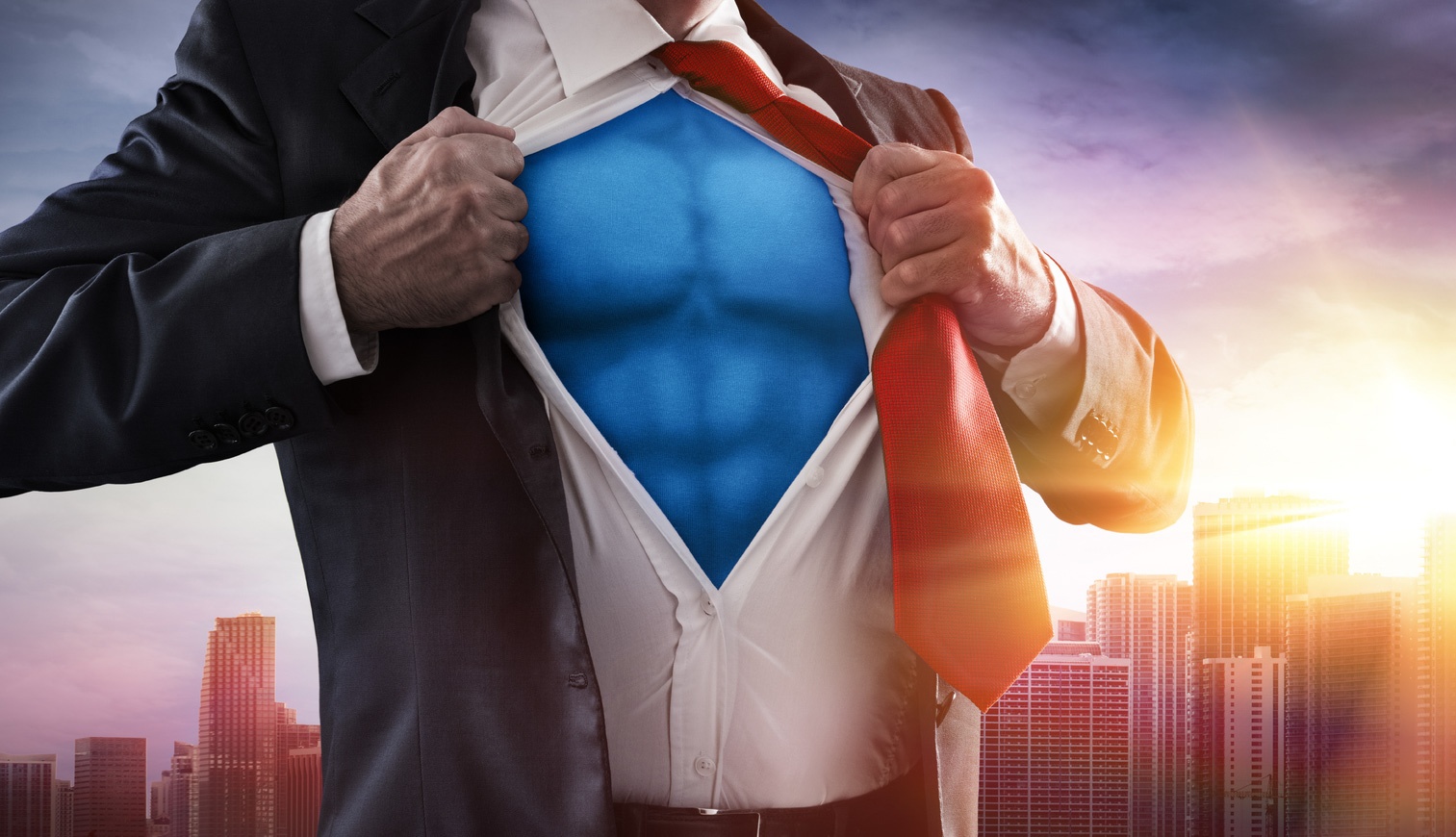
Most people have never heard of causal learning, but the concept is an important one for the future of healthcare because it holds the key to understanding and fighting disease and improving outcomes. However, to understand how and why causality is so important in the fight for better healthcare, we have to first understand machine learning.
You may be familiar with the term “artificial intelligence”, which is a broader umbrella term that machine learning falls within. An article in Xconomy published earlier this year goes into detail on artificial intelligence (AI) and starts by talking about the bots we are all becoming familiar with – Siri, Alexa, Cortana, etc. – but makes the case that the real action and future in AI or machine learning is “Headless AI”. As the author explains it:
“Headless AI combines machine intelligence and learning loops to constantly evolve. Because these solutions plug into the data lifeblood of a company, they become incredibly valuable as the algorithms adapt to the patterns that work. I call this form of AI “headless” because, unlike bots, the value is mostly not about the personality. Headless AI works with humans and augments their strengths; it doesn’t try to replace them. It gives people superpowers.”
Since computers are now capable of looking at models and data sets so big that human brains literally cannot process them on their own, being able to connect that learning to the human mind in a way that is useful is the future of machine learning. These causal models of the mechanisms of a system explain how the system works which is critical for healthcare. At its core, this type of AI is what GNS Healthcare – and their REFS (Reverse Engineering – Forward Simulation) platform – excels at.
Bruce Church, the Chief Mathematics Officer at GNS helped explain machine learning and what it is doing to revolutionize healthcare. Church is a self-professed “evangelist” of making machine and causal learning easy to understand. “Traditionally, computers have been programmed to solve problems in a linear fashion. Step one to step two, and if ‘this’ happens, then do ‘that’ until the steps are completed. It is a rational, manipulative way to complete tasks and has worked well for us for decades. Machine learning systems, however, are based on programs that solve an entire class of problems instead of just a single problem,” Church told me. “It can look at data in minute detail or on a global scale and it is not fixed in its approach, but is flexible by design. These programs can be trained with data to find the parameters and insights that humans would not be able to identify on their own, but need to consider in order to find new answers.”
Causal Learning Excels at Modeling and Understanding Incredibly Complex Systems
Church gives a great example to explain how machines look at data. “When humans look at a picture, we see an elephant. However, when a computer looks at the same picture, it sees millions and millions of individual pixels. If humans were to look at the individual pixels, we would never know what the image was, but with machine learning, computers can identify edges, borders, areas of differentiation between the different pixels and provide insight on a granular level that humans may have missed. It might not tell us that it is an elephant, but it can absolutely identify subtle areas of interest that we would have overlooked.“
As machine learning approaches get smarter, deep neural networks are starting to excel at classifying images and other data ls and humans are starting to purposefully step back and let machines do their thing. “Some machine learning approaches are powered by humans putting their preconceptions on a system,” says Bruce. “This is a glioblastoma tumor, this is what a border looks like, they are usually shaped like this. Machines can excel at this type of analysis, but what if we told the AI, just forget about how a doctor or a philosopher would think and just look at the data, analyze the data and you tell us what it says. These approaches are leading to real breakthroughs.”
Causal learning takes all of this one step further, by allowing humans to peer into a mechanistic model of a system and ask questions about why something happened or what ‘will happen if?’.
For example, aerospace engineers use causal modelling all the time. They know the size of the wing on their airplane or spaceship and can use causal modelling to determine if their assumptions about the physics and dynamics of airflow and thrust are correct. They can ask “If I change the shape of this wing, what will happen when we go supersonic? Engineers do this by hand – with the help of computers – on a daily basis, because we understand the physical models so well. It is amazing to think that aerospace engineering is child’s play compared to biological systems,” says Church.
Causal learning connects the worlds of data mining to mechanistic modeling and is what is required when a modeled system is just too complex for humans to assess without machines doing most of the heavy lifting. Using computers to look at an entire disease or how a particular drug will perform for individual patients requires complex algorithms and models that are just now being appreciated in the healthcare market at large, but which GNS has been delivering on for years. According to Church, the ability to gather and integrate genomics data, healthcare records, details about previous interventions, subpopulation insights and scores of other types of data into a single cohesive model that can be interrogated, sets the table for causal machine learning to help people make the shift from a ‘what do I see?’scenario to a ‘what do I do?’ mindset.
Causal Learning Connects Machine Learning To Humans to Deliver Optimal Interventions and Business Value
In essence, causal learning is all about making better, more informed decisions that help deliver optimal healthcare interventions. It does that by allowing people (researchers, doctors, scientists, business decision makers) to reverse engineer and then to interrogate highly sophisticated models, take the details of what the machine has learned and shown them and ask ‘what if’ questions. As Church explained, you can build a model on huge amounts of data about a disease, then layer in data about how people respond to treatment, then put in all the information about their biology and the decisions physicians made about their treatments, then you can interrogate that incredibly rich model and find clinical markers that provide insights into who something will or not work for.
This creates a ‘what do I do” opportunity.
Causal modeling delivers the ability to define probability around the idea that this intervention will have the highest likelihood of working for this population, or subpopulation or person. As Church sums up, “It’s the benefits that are the point. Causal learning delivers insights and access to information about biological and physiological systems, diverse patients, that have never been possible before and it is allowing businesses, physicians and foundations to take those findings and turn them into better outcomes for patients.”