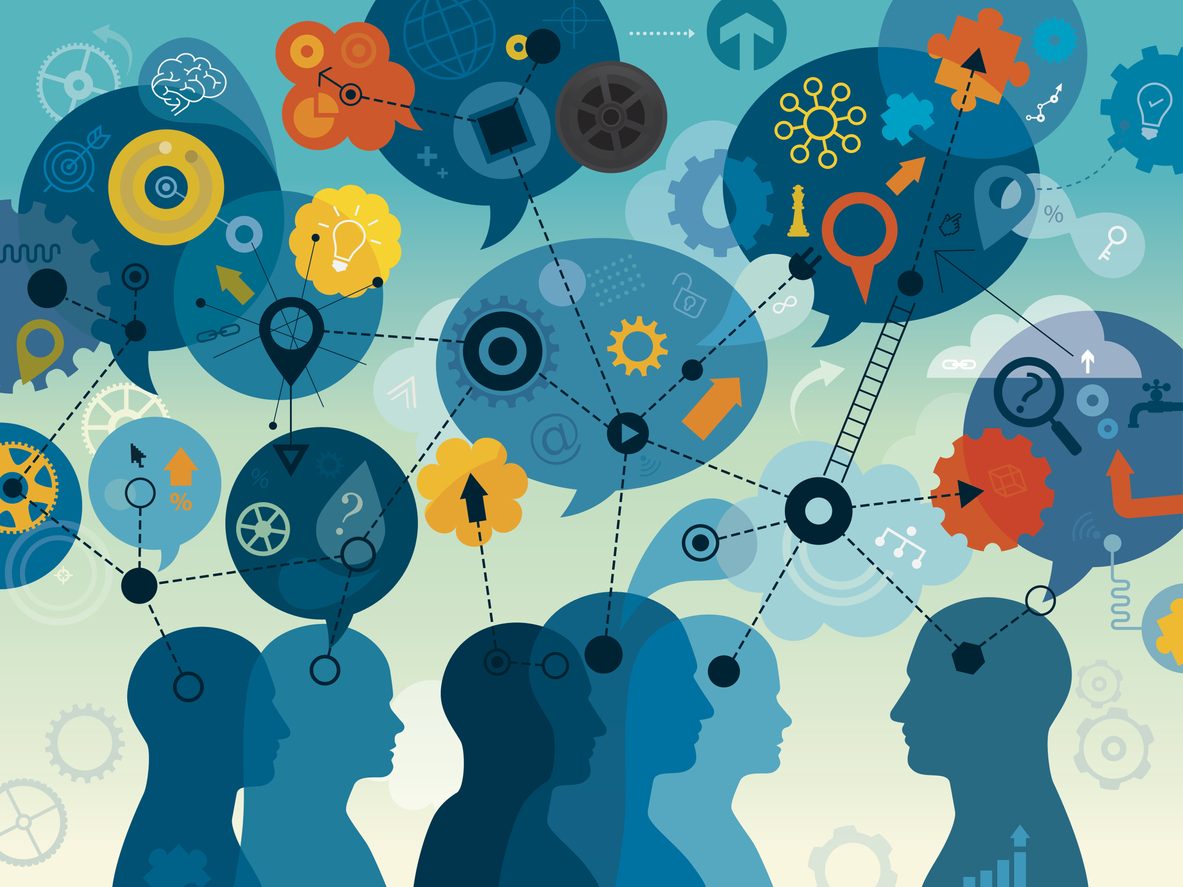
The following is the first in a three-part series on the impact of AI on the product development life cycle of drugs from discovery, to clinical trials and market access.
Biopharma companies and government funded research projects have produced stellar results over the years: from lifesaving drugs and to life improving therapies that benefit millions of patients. But these discoveries often come at a substantial financial cost and take many years to bring to market. In fact, the cost of bringing one drug from discovery to approval is currently estimated at between $1.8 and $3.0 billion.[1]
Unfortunately, despite the improvements in drug development, from basic science to preclinical and clinical development, the process of discovering new molecules and understanding the biological underpinnings of disease is becoming progressively more difficult. This is reflected in the number of new drugs approved – the number of drugs per billion U. S. dollars spent on R&D has halved roughly every nine years since 1950, decreasing nearly 80-fold in inflation-adjusted terms.[2] Drug discovery has become slower and more expensive over time, even with significant improvements in technology. In fact, data shows that the cost of developing a new drug has doubled every nine years.[3]
Why has Drug Discovery Become Increasingly Complicated?
A number of theories have been advanced to account for the downward trend in drug discovery versus dollars spent. Saturated markets, decreased risk tolerance from regulators, larger legal departments, and other factors may all play a part.
Few would disagree that it has become more complicated to develop new drugs. Complex diseases with multiple mechanisms of action often require combination therapies. This is particularly true in oncology and rare diseases but is evident in many other drug targets as well.
Discovering treatment options requires identifying the biomarkers implicated in disease progression and subsequently finding a working process by which to act on the disease process. This discovery process is a classic multivariate problem, except the variables ,in many cases, have yet to be discovered and are not optimized to work in a system that is still highly unpredictable: the human body.
Scientists are left simultaneously trying to understand underlying biology, which is complex machinery, and identify molecules which can perturb the biomarkers in this system. Biomarker-focused drug development is currently driven by two key trends: a push for biomarker discovery and validation in smaller, early-stage trials, and the explosion of data that can be generated via multiple ‘omics profiling technologies. At the intersection of these trends, the well-known “curse of dimensionality” means that there is a critical need for high performance mathematical algorithms capable of separating biomarker signals from the noise.
Unfortunately, conventional machine learning and biostatistical methods struggle to separate false-positives from true predictive and prognostic biomarkers, leading to low probability that hypothesized biomarkers will validate in prospective clinical trials.
How Artificial Intelligence Fits In
Researchers are constantly looking for ways to overcome the growing complications involved in discovering new drugs and many are coming to realize that AI can help solve the complex problems inherent in drug discovery.
While the amount of data collected, both in clinical settings and beyond, is steadily growing, not all data is created equal. In oncology development for instance, which accounts for roughly 40% of R&D and clinical trials and investigations, the number patients participating in clinical trials or even affected by a particular cancer can be fairly small.[4] When the sample size is small, and the number of potential biomarkers or predictors is large, sophisticated causal modeling and artificial intelligence is the best tool to tackle the complex datasets and provide biomarker insights for precision medicine.
The use of causal AI, rather than strictly time consuming and resource intensive lab experiments, can provide earlier biomarker identification, subpopulation segmentation, and the possibility of putting small Phase I or II trials to more effective use.
Access to rapid model testing solutions also streamlines the work companies are doing while conducting trials for the same molecule with several different applications. Rather than waiting for results, data can be continuously added to the mechanism, allowing algorithms to dynamically learn and adapt, providing new insights faster. Rather than rigid silos, AI can exploit multiple data streams concurrently and generate far more impact from each result. Fundamentally, AI can evaluate the potential of molecular candidate much faster, and perhaps better, than humans. Working together – clinical researchers and data scientists can leverage these tools for stronger, faster, more affordable impacts. So, if you ask us, we think AI and drug discovery make the perfect partners to better understand biology, how diseases progress and which patients respond to drugs.
To learn more about how drug development can be transformed with AI, download our eBook.
Stay tuned for the second piece in our series on AI’s impact on Clinical Trials.
[1] A Paradoxical Trent in Drug Development, by Hesam N. Motlagh, Atana-Medium, January 23, 2018
[2] Diagnosing the decline in pharmaceutical R&D efficiency, by JW Scannell, A. Blanckley, H. Boldon, B. Warrington, NCBI, PubMed.gov, March 1, 2012.
[3] Moore’s and Eroom’s Law in a Graph – Skyrocketing Pharma R&D Costs Despite Quantum Leaps in Technology. Blaint Botz – Medium, September 13, 2016.
[4] Innovation in the Biopharmaceutical Pipeline, PhRMA