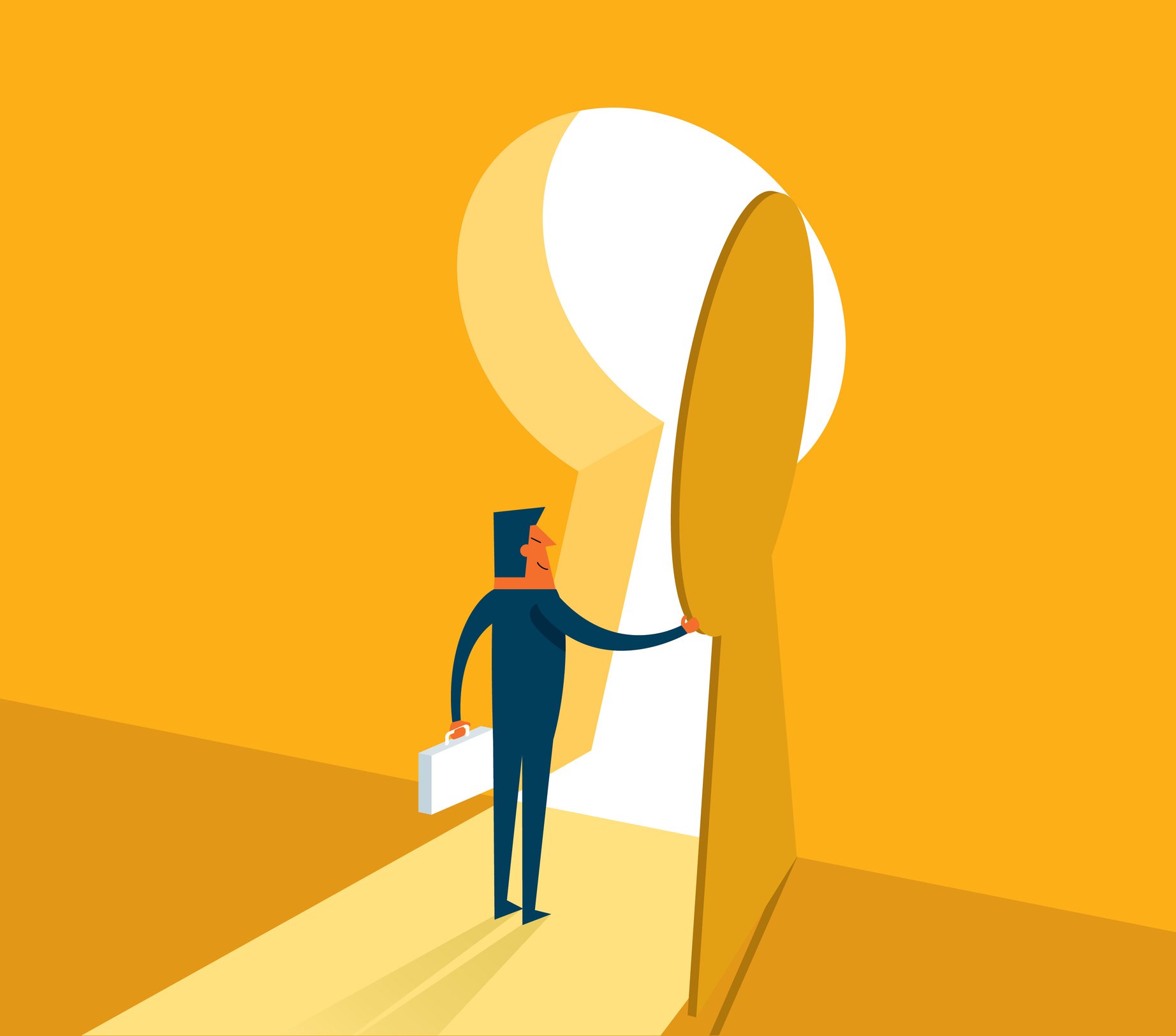
This is the third in a three-part series on the impact of AI on the product development life cycle of drugs from discovery, to clinical trials and market access.
Regulatory approval of a drug does not immediately translate into premier market access or projected results. The reality is that the pressure on pharma companies from health plans and pharmacy benefit managers (PBMs) to provide evidence-based research on treatment efficacy and effectiveness before including drugs on their formularies is only increasing.
The earlier pharma companies can answer these asks, the better. The goal is to bring better drugs to market faster and drive towards personalized medicine by identifying which treatments work best for which patients. By analyzing real-world data and leveraging AI, pharma has the tools needed to better meet these demands.
The challenge of market access
Market access is the idea that the patients who would benefit from a treatment can receive rapid, and sustained, access to the solution they need. The challenge however, is that individual stakeholders across the healthcare spectrum, payers, pharma, and providers have a diffuse set of standards and processes by which to provide these treatments. As the healthcare industry relies primarily on randomized clinical trials (RCTs) and traditional statistical methods to extrapolate results, the process of effective personalization is often difficult. With the power of AI however, identifying the right subpopulations for effective response, even after a drug has been brought to market is not only possible, it’s the next frontier.
When researchers incorporate real-world data (RWD) into their studies to analyze the true impact of the drugs they are developing the results can provide the proof of value necessary to achieve robust market access.
The role of AI
AI enables researchers to leverage real world data and evidence to better understand the mechanisms of action and uncover key drivers of disease progression. This provides pharma companies with insight into how the drug will perform in the real world, and provides the evidence needed for targeted adoption in the marketplace. If market access strategy is reframed as patient access strategy, then RWD being used to meet the demands of patient trial recruitment becomes very useful both to market access and R&D teams.
Providing proof of efficacy
To ensure the commercial viability of products, pharma companies must consolidate and analyze vast amounts of disparate structured and unstructured RWD and extrapolate valid conclusions quickly. AI and machine learning help define and prove the effectiveness of drugs by fostering a clear understanding of what outcomes matter and what is driving those outcomes biologically. This work is important not only in comparing RWD from specific drugs to each other, but also to non-drug-based treatments which can be the current standard of care.
Targeting responsive subpopulations
Causal machine learning provides a transparency- often called white box AI- that traditional statistical analysis and predictive solutions cannot, as it identifies which variables are driving outcomes and most importantly why. The advanced computing power of causal machine learning enables researchers to conduct a greater number and wider variety of in silico trials with large data sets to discover drug indications, identify patient subpopulations that respond and predict outcomes earlier in the process. This means that companies can adopt a much more precise approach, focusing their efforts on patients who will respond well, potentially saving money throughout development and commercialization processes.
Track patient journeys
Using longitudinal RWD, pharma companies can construct and aggregate patient journeys to discover co-occurrence patterns for different regimens, tests, diagnoses, treatments, adverse events and the length of duration. This has historically been a monumental task, one that can now be tackled with AI as a primary data analytics and forward simulation technology. With AI, historical patient journeys can be used to simulate the realities of future patients, accelerating the selection of proper treatment protocols which patients are more likely to tolerate. The crucial insights generated through causal AI not only help companies better understand their drugs, but also prove their value for the right patient populations.
The task of market access teams, minimizing the barriers patients face in obtaining the therapies they need to live a healthier life, is by no means a simple task, but effectively leveraging causal AI throughout the drug development and commercialization process can be transformative.[1] What better way to lower the barrier than identify exactly which patient subpopulations would benefit from a treatment most, as early as possible in the development process?
To learn more about how AI can help with market access and commercialization for pharma products, download our eBook.
[1] Repositioning Market Access: A Function Fit for Purpose in a New Era of Costly Cures