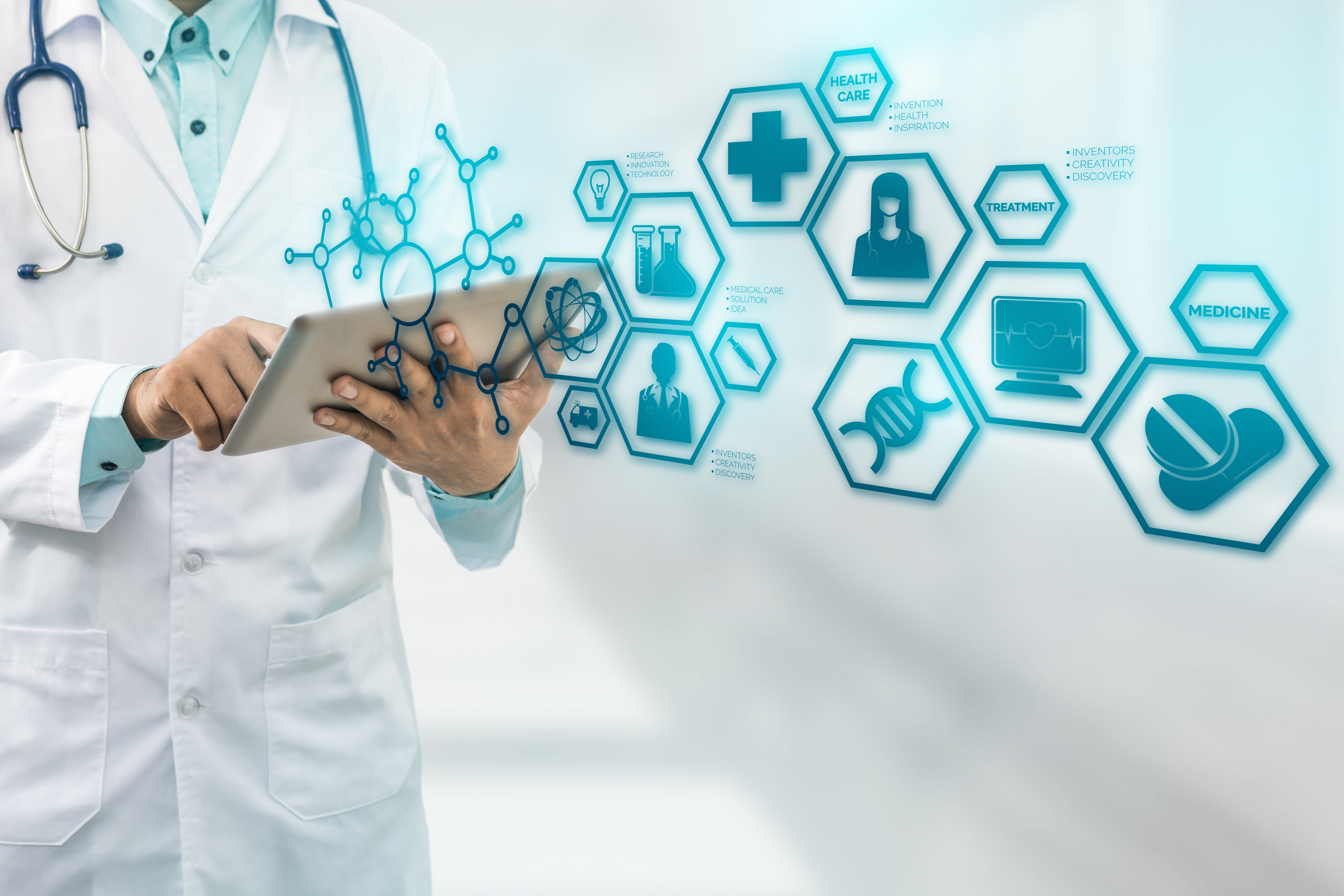
By Patrick Getzen, Chief Data and Analytics Officer of Blue Cross and Blue Shield of North Carolina
Clashes between the traditional and the novel are as old as time itself. Change is rarely welcomed, so anything new that comes along often faces a rough road to acceptance. However, that is not the case when it comes to the types of data that are now becoming available in the world of healthcare. The merging of new and traditional healthcare data promises to lead to a new era of discovery and offers significant benefits for patients, providers and payers.
Expanding data sets, expanding patient knowledge
Thanks to Meaningful Use, we have reached nearly 100 percent implementation of electronic health record (EHR) systems in healthcare organizations. This has provided wide availability of traditional sources of payer data such as claims, wellness and other healthcare program activity-based information, such as disease and case management.
While these data sets are crucial as we attempt to navigate the new world of value-based care, it is combining this traditional data with emerging data types and data sets including social determinants of health, sensor, wearable, medical device data, enhanced EHR and genomic data, that holds the promise of exciting new breakthroughs in care treatments.
Social determinants of health are conditions in the environments in which people are born, live, learn, work, play, and age that affect a wide range of health, functioning, and quality-of-life outcomes and risks. Social, economic and physical conditions and settings in these various environments can dramatically impact health outcomes.
Wearable devices make it possible for physicians to monitor a patient’s condition far-removed from the examination room. Providing this real-world data to researchers opens new doors in determining the effectiveness of therapies.
Genomic data, now rapidly available, holds the key to precision medicine – matching the right therapy to the right patient at the right time.
While not fully proven yet, the idea is that these new data types enable a more holistic view of patient health than traditional data sources. The applications and opportunities are broad and varied, ranging from predictive analytics for earlier and more impactful care management opportunities, to reducing barriers to healthcare.
One example of an interesting application: The Blue Cross and Blue Shield Association has recently partnered with Lyft to provide transportation to medical appointments for those who lack transportation (as identified through social determinants of health data).
In addition, we have interest in real-time or near real-time consumer “touch” or interaction history that allows for more personalized engagement and better decision making to facilitate navigation within the healthcare ecosystem. The application of next best action capabilities leveraging this data is of great interest.
Machine learning key to unlocking new insights
Understanding how to optimize clinical pathways at a personalized level will be pivotal for improving quality of care and driving affordability in the healthcare system. Intersecting clinical pathways with consumer engagement information is arguably the holy grail of machine learning and artificial intelligence in healthcare.
Two of the biggest challenges are the overwhelming complexity in the healthcare system and the fragmented data it generates. The increased computer power that is now available allows us to rapidly analyze this data. Machine learning takes advantage of these two developments – enormous computing power and growing availability of data from multiple sources – to reduce medical expense and improve customer experience in ways that were not possible just a few years ago.
Causal analysis as well as machine learning lend themselves to simulating randomness in machine learning algorithms to determine causality.
Partnering with a third party may be the best way to take advantage of this powerful technology. Augmenting AI and machine learning makes sense when there is a technology, functionality and/or capability that can be provided cheaper, better and faster than in-house development. We have an interest in platforms that have broad application across functions and processes throughout the enterprise, where historically we would have had multiple, disparate solutions.
However, it is not enough for a partner to provide machine learning insights from a ‘black box’ solution. They must provide the supporting information that makes the insights actionable. For example, a predictive model that identifies members at risk for becoming a high cost member in the future is only helpful if there is insight into the modifiable factors that influenced the risk. Causal models provide this depth of insight unlike correlation models.
Enabling technologies for machine learning
Core enabling technologies for AI are platforms that enable ease and flexibility of data sourcing (i.e., structured, unstructured, semi-structured). Additionally, platforms that leverage advances in machine learning and artificial intelligence are equally as important.
We have seen some early successes through the use of advanced technology. We’ve built advanced models in avoidable emergency room visits, hospital readmissions and pharmacy outliers (such as prescribing a medication with a lower-cost equivalent).
Investing in machine learning for value-based care
Causal machine learning, a powerful form of AI, shows huge potential in helping us transform the way healthcare is delivered. As the volume, quality and types of data increase, healthcare stakeholders’ challenge will be to use the best methods to unlock value from that data. Applying artificial intelligence and machine learning to this ‘big data’ can lead to optimized interventions that drive better care outcomes at lower costs – key goals for those of us committed to the goal of personalized medicine.