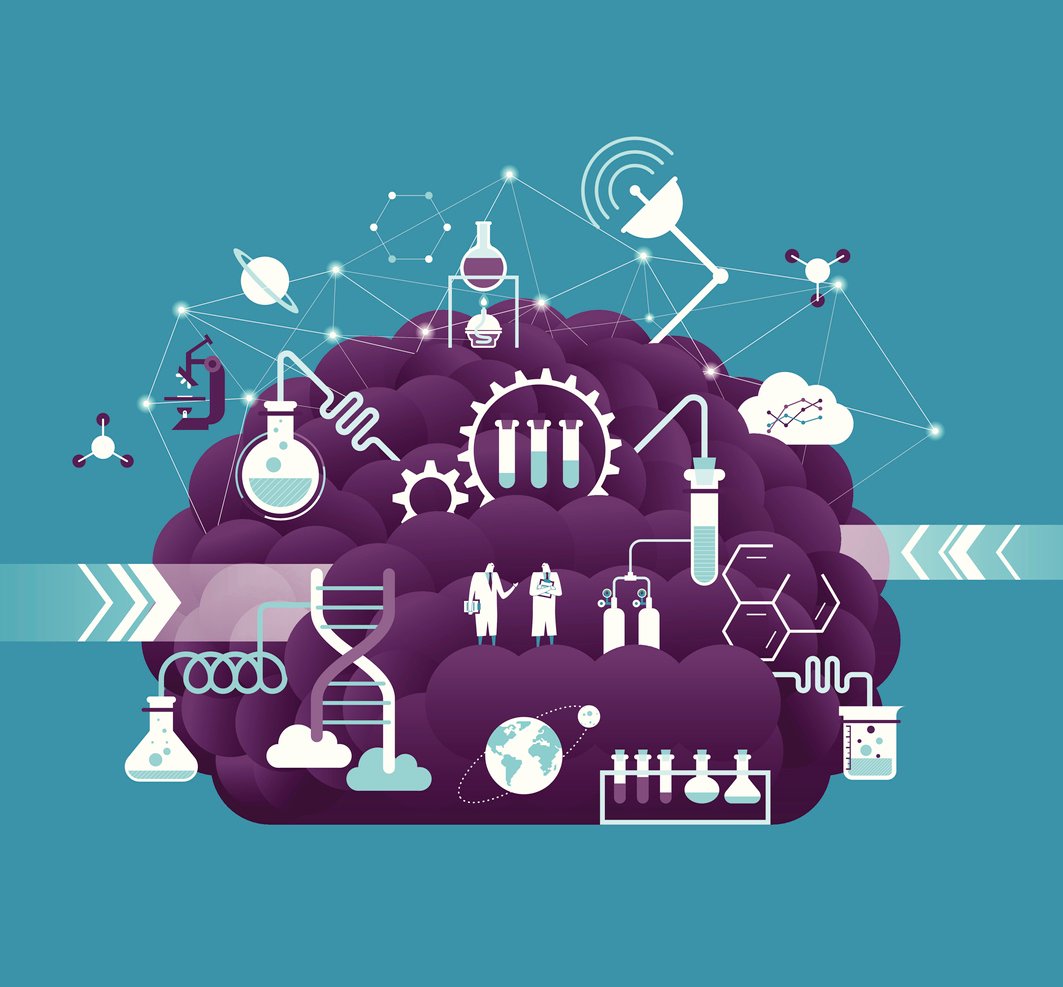
The following is the second in a three-part series on the impact of AI on the product development life cycle of drugs from discovery, to clinical trials and market access.
The success of clinical trials relies heavily on having enough of the right patients willing and able to participate. Unfortunately, achieving the levels of recruitment necessary to conduct successful trials is difficult – particularly in cancer and rare diseases trials.
One recent survey revealed that only one in three cancer patients were likely to enroll in a clinical trial. Less than half of those surveyed viewed clinical trials favorably and 25% saw participating in a trial as a “last resort” for treatment. Other reasons for unwillingness to participate include concern over unproven treatments, safety and side effect issues, lack of insurance coverage and general inconvenience.[1]
Finding patients willing to enroll in a clinical trial is only the beginning. Getting them to stay in the trial to the end is equally challenging. A study on patient retention revealed that the average dropout rate across all clinical trials is 30%.[2]
Not recruiting enough of the right patients or not being able to prevent dropouts is becoming an increasingly critical issue in the clinical trial process. Half of all clinical trial recruitment sites enroll one or no patients. Eighty-five percent of clinical trials fail to retain enough patients and as a result 80% of all clinical trials fail to finish on time. Each day a trial is delayed can cost pharma trial sponsors between $600,000 and $8 million.[3]
Fortunately, it’s a new world. The application of AI for clinical trials can impact patient recruitment and retention strategies, protocol design, and break down data silos for more efficient across multiple sites and simultaneous trials.
Identifying Patient Subpopulations for Clinical Trials
Artificial Intelligence (AI) is a key tool to solving the dilemma of identifying and recruiting the right patients for clinical trials, by providing analysis to guide the refinement of clinical inclusion/exclusion criteria to make it easier to find the right participants.
Currently, there are nearly 20,000 clinical trials open for patient recruitment. Ninety percent of them will be delayed because of a lack of enrolled patients. A third of those will eventually be suspended for the same reason.[4] Insights gained from these trials is critical and highly dependent on streamlined recruitment efforts.
Traditional recruitment strategies still rely heavily on physician recommendations and proactive outreach from trial sites. However, recruiting the right patients starts with understanding specific subpopulation biomarkers to ensure the right patients are being recruited – those who are most likely to respond to a drug. For this process to work effectively, it’s important to establish which biomarkers a drug is acting on, which can be done in the drug discovery phase as discussed in our previous blog post.
With the help of AI tools the approach to recruitment changes. Rather than recruiting patients on broad characteristics, rigorous AI solutions can pinpoint the predictive and prognostic markers evident in a subpopulation which can not only inform the development process, but could also refine exclusion and inclusion criteria for trials. For instance, in work done by GNS Healthcare and the Michael J Fox Foundation, a subset of Parkinson’s patients were identified as having faster disease progression. In the study published in The Lancet, authors showed that patients with specific alleles show faster rates of motor decline. A major challenge for Parkinson’s Disease drugs and trials is that progression rates are extremely varied, making it difficult to include the right patients in appropriate trials. With the use of AI and biomarkers to find accurate predictors of progression rates the degree of uncertainty in development and trial protocol can be mitigated. Selecting for participants using refined specifications can not only reduce the number of participants required but increase the probability of success of the trial and lower dropout rates.
Breaking Down Data Silos
One of the primary challenges in the clinical trial process is how the data that is collected is used. Often achieving one primary endpoint triggers the decision to move to the next phase of a trial. The next question should be what can be learned from the rest of the data? Even though the study may have met the statistical criteria established for the primary endpoint, other data from the study unrelated to that specific endpoint might provide contradictory information that would indicate a false discovery. Researchers need to be sure they are analyzing all the data to ensure that the results are valid. There is a clear need for novel methods to analyze data differently and faster. If the process is too lengthy, decision-making is delayed, and the final conclusion may not include all the analysis necessary.
The future of clinical trials may well be AI driven, with subpopulations identified by algorithms driving better trial protocol design and reducing the number of patients required for a successful trial. So yes, it’s a new world for drug development, one that will better match drugs to patients and speed treatment to those who need it.
[1] 65% of Patients Unlikely to Volunteer for Clinical Research, by Jennifer Bresnick, HealthIT Analytics, May 24, 2016.
[2] Retention in Clinical Trials – Keeping Patients on Protocols, by Kristina Lopienski, Forte Research, June 1, 2015.
[3] How Artificial Intelligence is Transforming Clinical Trial Recruitment, by Iman Ghosh, Visual Capitalist, October 25, 2018.
[4] Meet the Company Trying to Democratize Clinical Trials with AI, by Megan Molteni, Wired, January 30, 2018.