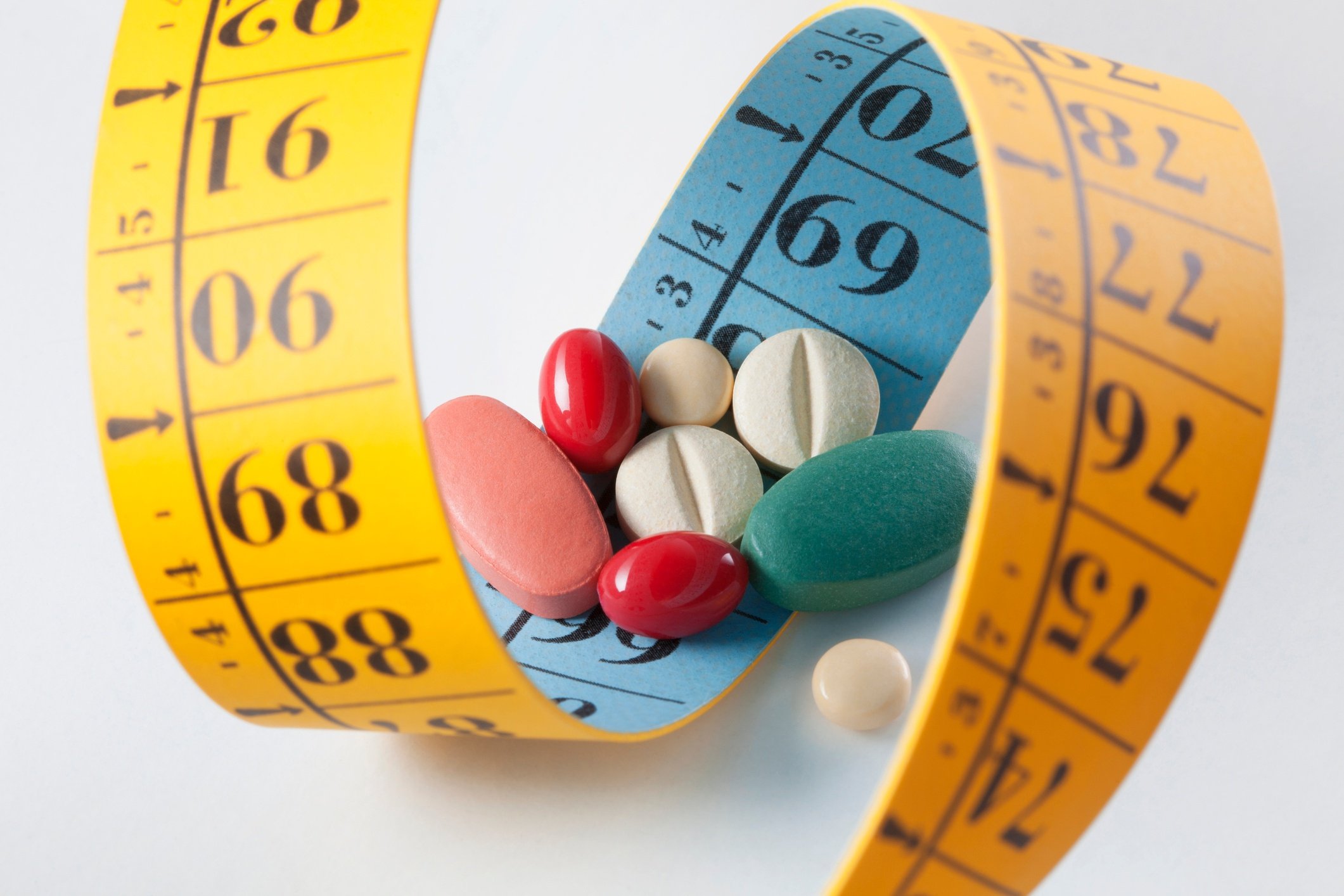
In the summer of 1996, two new prescription drugs, containing fenfluramine and phentermine, hit the market promising an easy way to lose weight. Use of the products skyrocketed and by mid 1997, almost two million people were taking them¹.
Just over a year later, in September of 1997, both products were abruptly pulled off the market amid growing concerns that use of the drugs may cause serious heart problems. The FDA found evidence that nearly one-third of those taking the drugs might develop a rare form of heart valve damage that could weaken the heart. Three deaths were linked to the use of the drugs².
Something didn’t make sense. The drugs were approved by the FDA based on results from randomized controlled trials (RCTs). However, once the products were being widely used, it became clear that they could potentially cause severe problems. This case highlights one of the key challenges in drug development: results from RCTs don’t – and can’t – always replicate real-world results. Since data is quickly becoming more available, it’s time to leverage the power of real-world data earlier in the drug development process.
The limitations of RCTs
Despite the serious drawbacks illustrated by the weight loss drug case, RCTs have traditionally been the sole method of determining the safety and effectiveness of proposed drug therapies. Researchers now understand that the highly selective patient cohorts and controlled environment of RCTs are not able to replicate conditions that result when a larger, more diverse population is exposed to a drug.
To mitigate this problem, researchers are now incorporating real-world evidence (RWE) into their studies to analyze the true impact of the drugs they are developing. RWE includes diverse and large volumes of data from electronic health records (EHRs), insurance claims, genomics, social media and wearables that provide information on real patients using the medication. This gives pharma the ability to track a patient’s long-term progress and response to the drug. This crucial information can be enormously beneficial during all stages of drug development.
How RWE can make a difference
Why is the use of RWE so important when it comes to drug development? Because there are many things RWE can help reveal about drugs going through the development process that are simply not possible with RCTs. For example, even after FDA approval, physicians often don’t know the optimal dose, ideal length of treatment or which patients are most likely to respond to the drug. They also don’t know which patients may experience an adverse reaction to the medicine.
RCT’s focus on safety and efficacy, but all the key stakeholders in the healthcare industry – biopharma companies, health plans, providers and patients - want to know more about the drugs that are being approved. RWE helps uncover additional information like comorbidities, non-adherence and other variables that are not revealed in a controlled RCT.
The strength of RCTs is that they offer a standard process with strictly monitored conditions to develop medical therapies. The weakness is that these trials involve highly targeted, homogenized populations that will not reflect reactions to the drug in the real world. For that type of information, researchers need RWE.
Government getting on board
The FDA is beginning to recognize the importance of RWE in drug development and on July 18th, it finalized guidelines detailing a policy for using EHR data in FDA-regulated clinical investigations. According to the guidelines, pharma companies can now use conclusions drawn from EHR data to support the approval of new indications for already-approved drugs or to fulfill study requirements after approval.
The release of the guidelines comes as a result of a mandate in the 21st Century Cures Act passed by Congress in 2016 that requires the FDA to evaluate the use of RWE in the clinical trial process. The Prescription Drug User Fee Act VI passed in 2017 also enhances the FDA’s ability to consider the possibilities of using “real world” data as an important tool in evaluating drug safety and efficacy. The FDA is now holding public forums to address concerns in the use of RWE for regulatory decision making.
Leveraging RWE with AI
Making RWE available for researchers is a solid first step. The next hurdle is determining how to analyze the data to uncover insights that support drug development. The problem is that so much data are being created, researchers need new tools to process it fast enough to make it usable.
The enormous computing power of AI and machine learning can quickly absorb the increased variety, velocity and volume of RWE to discover the insights needed for faster drug development and label expansion. The explosion of RWE data dramatically increases the number of variables that can be examined. This introduces an analytic challenge that can’t be met using traditional statistical techniques.
The advanced computing power of causal machine learning enables researchers to conduct a greater number and wider variety of “in silico” experiments with large RWE data sets to discover drug indications, identify patient subpopulations who respond and predict outcomes earlier in the process.
According to a recent survey of biopharma leaders, 60 percent reported they are currently using machine learning to process RWE and almost 95 percent expect to incorporate it in the future³.
By marrying the power of RWE with advanced machine learning, biopharma now has the ability to unearth how their drugs are likely to fare with real patients in real world settings. This insight is crucial to not only understanding their drugs, but necessary to proving value, accelerating clinical trials and decreasing the cost of bringing a drug to market. It also advances personalized medicine so patients receive medications that are most effective for them as individuals. It’s a win-win for all involved.
To learn more about how data and artificial intelligence can improve the probability of success in drug development, download our most recent eGuide.
[1] 2 Diet Drugs Are Pulled Off Market, by John Schwartz, The Washington Post, September 16, 1997.
[2] Ibid
[3] The Future of real-world evidence, by Brett Davis, Jeff Morgan, Sonal Shah, Deloitte Insights, June 28, 2018.